A Deep Dive Into AI Cognition: Understanding Its Capabilities And Limitations
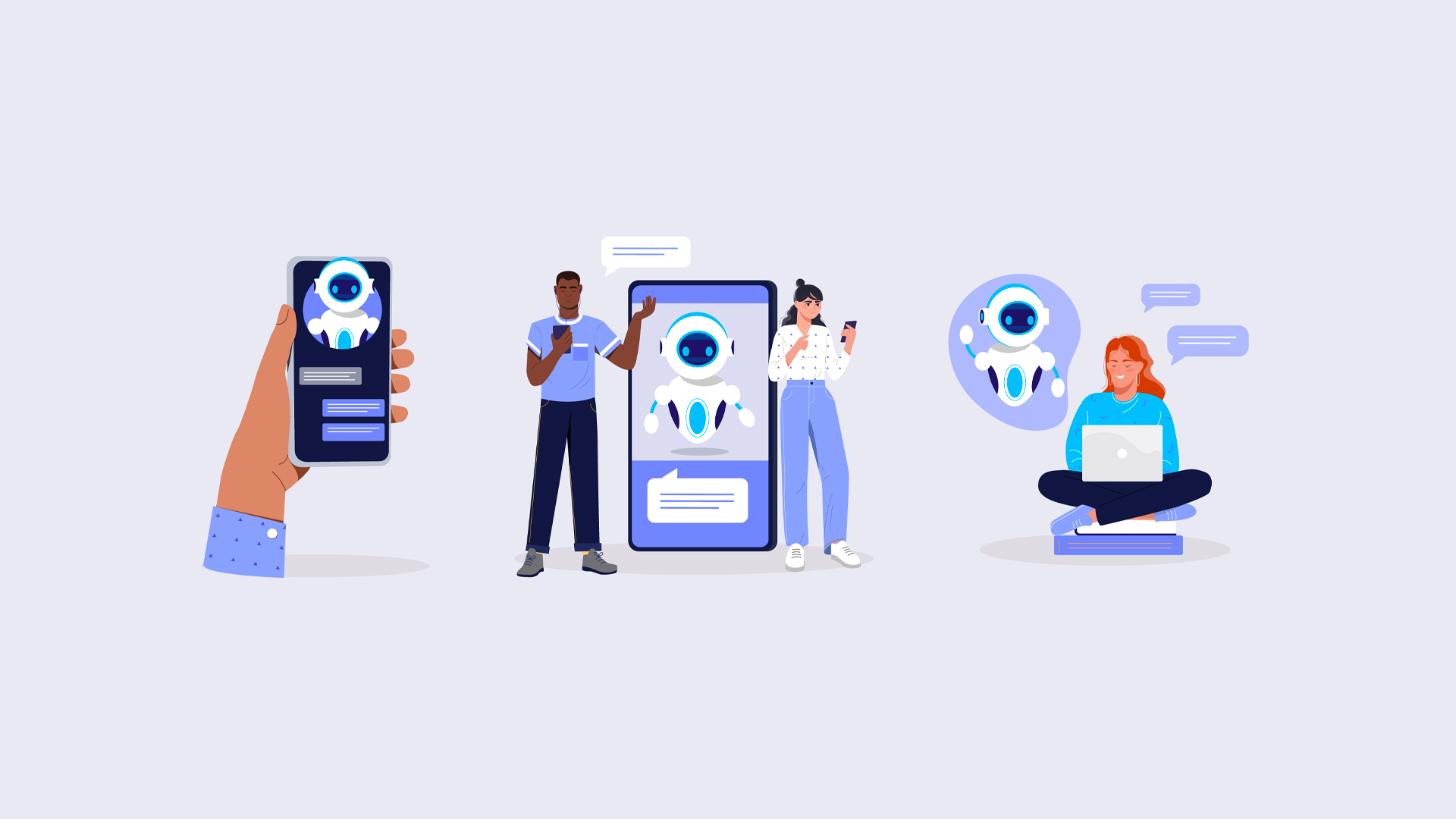
Table of Contents
The Capabilities of AI Cognition
AI cognition is no longer a futuristic fantasy; it's a tangible reality impacting various sectors. Its capabilities are constantly expanding, pushing the boundaries of what's possible.
Problem-Solving and Decision-Making
AI's prowess lies in its ability to process and analyze massive datasets far beyond human capacity. This allows for the identification of complex patterns and relationships, leading to effective problem-solving and decision-making in diverse fields. For instance, AI algorithms excel in medical diagnosis, analyzing medical images and patient data to detect diseases with remarkable accuracy. In finance, AI-powered systems predict market trends and manage investment portfolios more efficiently than traditional methods. The success of AlphaGo, an AI program that defeated a world champion Go player, demonstrates the power of AI in tackling complex strategic games requiring nuanced decision-making. These achievements are powered by sophisticated machine learning algorithms, deep learning networks, and reinforcement learning techniques, which enable AI to learn from data, adapt to new situations, and improve its performance over time.
- Superior speed and efficiency: AI can process information and solve problems significantly faster than humans in specific domains.
- Handling complex, multi-variable problems: AI excels at analyzing intricate datasets with numerous interacting variables, identifying patterns humans might miss.
- Potential for unbiased decision-making: When trained on unbiased data and with carefully designed algorithms, AI can minimize human biases in decision-making processes.
Natural Language Processing (NLP) and Understanding
Natural Language Processing (NLP) is a branch of AI focused on enabling computers to understand, interpret, and generate human language. Recent advancements have led to significant improvements in AI's ability to interact with humans naturally. Chatbots provide instant customer support, language translation tools bridge communication gaps across languages, and sentiment analysis tools gauge public opinion from social media posts. These capabilities are driven by breakthroughs in transformer models and large language models (LLMs), which can process and understand the context and nuances of human language with increasing accuracy.
- Improved accuracy in language translation and comprehension: NLP models are achieving near-human levels of accuracy in translating languages and understanding complex textual information.
- Enhanced human-computer interaction: Conversational interfaces powered by NLP are making technology more accessible and user-friendly.
- Understanding context and nuance: Advanced NLP models are increasingly adept at interpreting the subtle meanings and context within human language.
Computer Vision and Perception
Computer vision enables AI systems to "see" and interpret images and videos, much like humans do. This technology powers object recognition in self-driving cars, facial recognition systems for security purposes, and medical image analysis for disease detection. Convolutional neural networks (CNNs) and image segmentation techniques are crucial in enabling AI to identify objects, understand scenes, and extract meaningful information from visual data.
- High accuracy in object and scene recognition: AI systems can now identify objects and scenes in images and videos with impressive accuracy.
- Wide range of applications: Computer vision is revolutionizing fields like healthcare, security, manufacturing, and autonomous driving.
- Handling complex visual scenarios: Ongoing research focuses on improving AI's ability to handle challenging visual scenarios, such as low-light conditions or occluded objects.
The Limitations of AI Cognition
Despite its impressive capabilities, AI cognition has significant limitations that must be acknowledged.
Lack of Common Sense and General Intelligence
Current AI systems primarily exhibit "narrow AI," excelling in specific tasks but lacking the general intelligence and common sense reasoning of humans. They struggle to transfer knowledge learned in one domain to another and often fail to grasp the complexities and nuances of real-world situations. The gap between narrow AI and Artificial General Intelligence (AGI) – a hypothetical AI with human-level intelligence – remains substantial.
- Knowledge transfer limitations: AI systems typically struggle to apply knowledge learned in one context to a different, even related, context.
- Holistic understanding deficit: AI lacks the holistic understanding of the world that humans possess, relying heavily on specific data inputs.
- Data dependency: AI models require vast amounts of training data to function effectively, limiting their applicability in data-scarce environments.
Bias and Ethical Concerns
AI systems are trained on data, and if that data reflects existing societal biases, the AI will inevitably perpetuate and even amplify those biases. This poses significant ethical concerns related to fairness, accountability, and transparency. For example, biased facial recognition systems may disproportionately misidentify individuals from certain demographic groups.
- Risk of perpetuating societal biases: AI systems can reflect and amplify existing inequalities if not carefully designed and trained.
- Importance of responsible AI development: Ethical considerations must be central to the development and deployment of AI systems.
- Need for regulations and guidelines: Establishing clear guidelines and regulations is crucial to mitigate the ethical risks associated with AI.
Explainability and Interpretability
Many AI models, particularly deep learning networks, function as "black boxes," making it difficult to understand how they arrive at their decisions. This lack of transparency hinders trust and accountability. Explainable AI (XAI) is a growing field focused on developing more interpretable AI models, allowing us to understand their decision-making processes and identify potential biases or errors.
- Challenges in understanding AI decisions: The complex nature of some AI models makes it difficult to trace their reasoning.
- Need for transparent AI models: Explainable AI is crucial for building trust and accountability in AI systems.
- Ongoing research in XAI: Active research is focused on developing techniques to make AI models more transparent and understandable.
Conclusion: Navigating the Future of AI Cognition
AI cognition represents a powerful force shaping our future. Understanding both its remarkable capabilities and inherent limitations is crucial for responsible innovation and deployment. While AI excels at specific tasks and offers significant advantages in various fields, addressing issues like bias, explainability, and the development of general intelligence remains critical. The future of AI cognition hinges on navigating these challenges responsibly. We encourage you to delve deeper into this fascinating field, explore its potential and limitations further through additional research, and contribute to the responsible development of artificial intelligence cognition, unlocking its transformative potential while mitigating its risks.
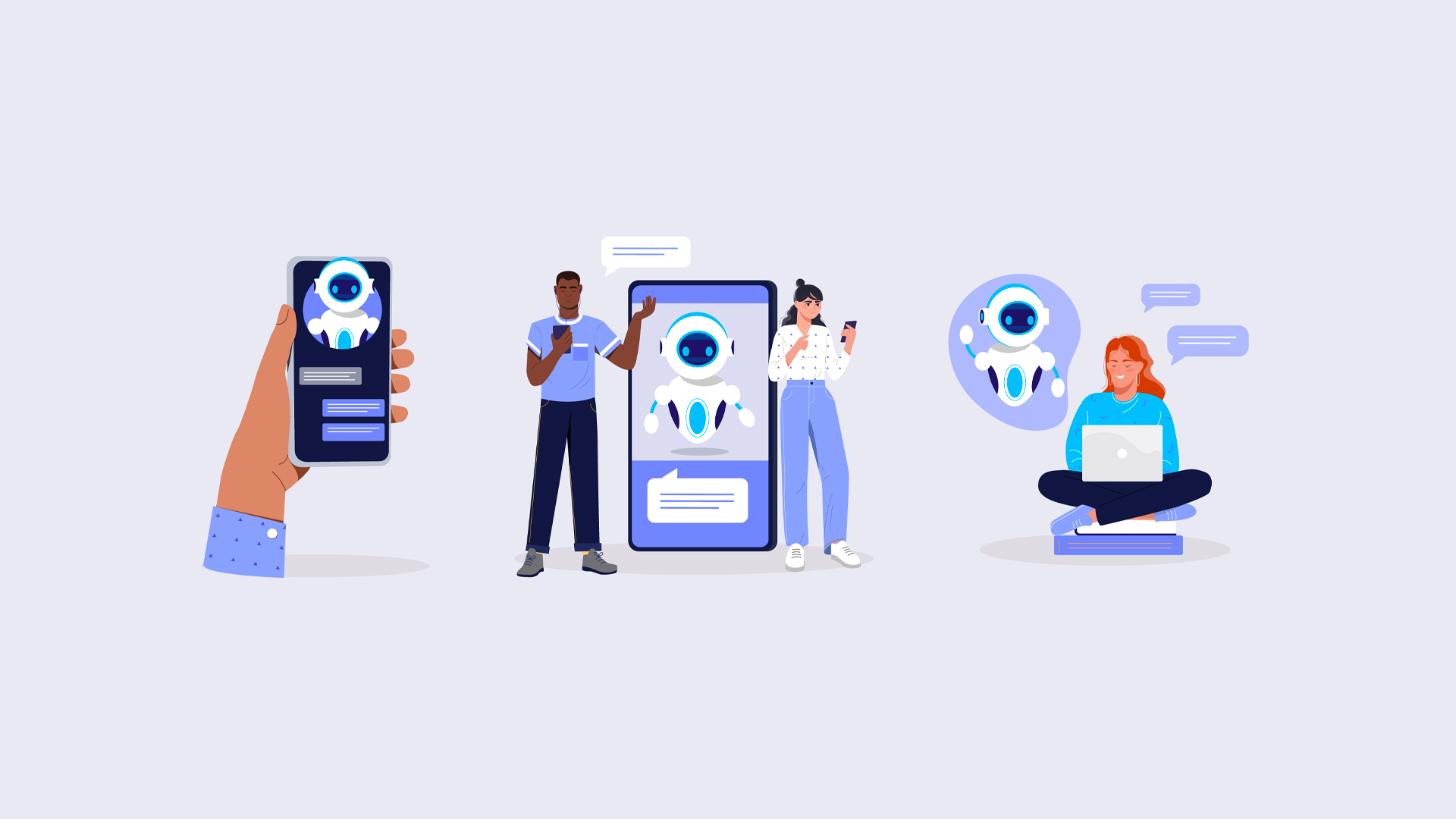
Featured Posts
-
Assessing The Risk Russias Military Actions And European Stability
Apr 29, 2025 -
Top India Fund Manager Dsp Sounds Warning Boosts Cash Holdings
Apr 29, 2025 -
Why Middle Managers Are Crucial For Company And Employee Success
Apr 29, 2025 -
Trumps Trade War Assessing The Impact Of Tariffs On Us Consumers
Apr 29, 2025 -
Evaluating Pitchers Name S Chances For A Mets Starting Role
Apr 29, 2025
Latest Posts
-
Kentucky Severe Weather Awareness Week Nws Preparations
Apr 29, 2025 -
Emergency High Levels Of Natural Gas Force Louisville City Center Evacuation
Apr 29, 2025 -
Fatal D C Black Hawk Crash Examining The Pilots Pre Crash Decisions
Apr 29, 2025 -
Firefighters Investigate Gas Leak Downtown Louisville Buildings Evacuated
Apr 29, 2025 -
D C Helicopter Crash Investigation Reveals Pilot Error As Cause Of 67 Fatalities
Apr 29, 2025