Accelerated Drug Discovery: D-Wave's (QBTS) Quantum Computing And AI Integration
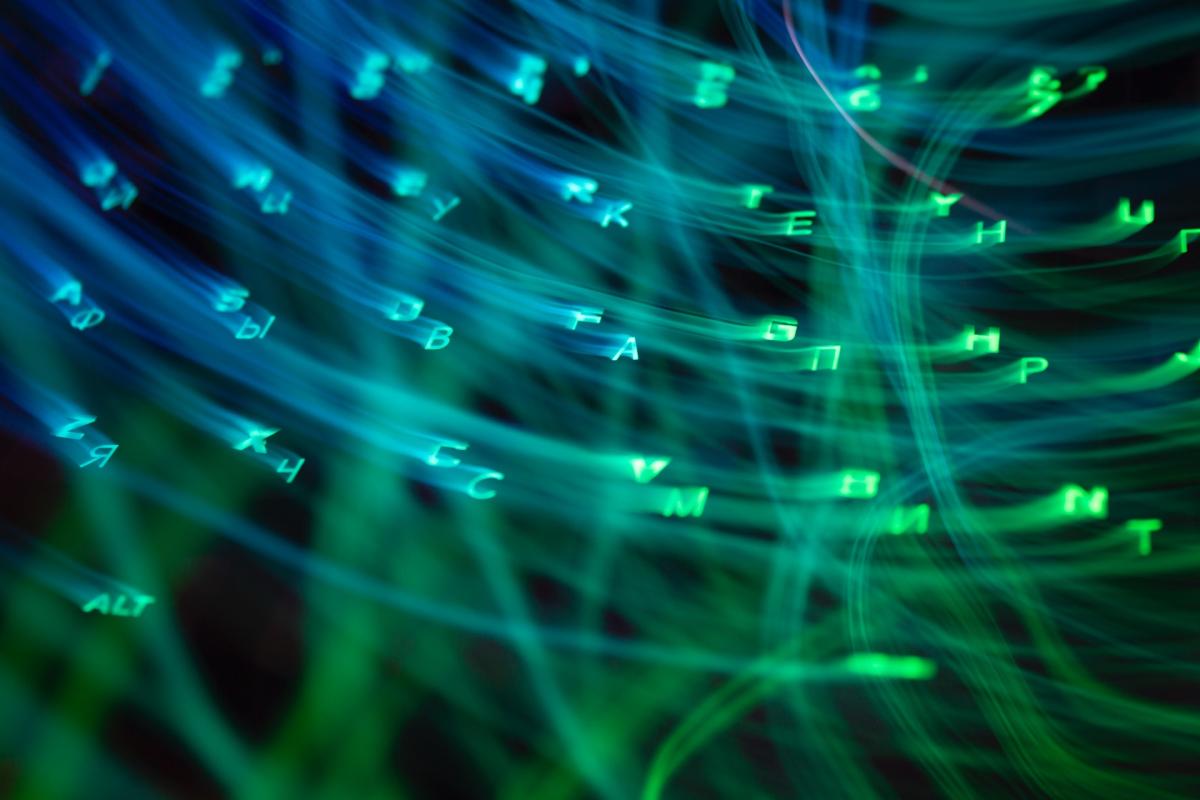
Table of Contents
The Power of Quantum Computing in Drug Discovery
Traditional computers struggle with the immense computational complexity inherent in molecular simulations and drug design. The sheer number of variables and interactions involved quickly overwhelm classical algorithms, leading to lengthy computational times and limited accuracy. Quantum computers, especially those leveraging quantum annealing like D-Wave's, offer a powerful solution by tackling these problems exponentially faster.
Overcoming Computational Barriers
The limitations of classical computing in drug discovery are substantial. Quantum computing offers several crucial advantages:
- Faster simulations of molecular interactions: Accurately modeling the complex interactions between molecules is crucial for understanding drug efficacy and toxicity. Quantum computers significantly accelerate these simulations, providing deeper insights faster.
- Optimization of drug candidate structures: Designing optimal drug structures with desired properties (e.g., high binding affinity, low toxicity) is a complex optimization problem. Quantum annealing excels at finding optimal solutions within vast chemical spaces.
- Improved prediction of drug efficacy and toxicity: Quantum simulations can enhance the accuracy of predicting how a drug will interact with the body, leading to more efficient pre-clinical trials and reduced development costs.
- Identification of potential drug targets: Quantum computing can assist in identifying promising biological targets for drug development by analyzing complex biological data and identifying key interactions.
Quantum Annealing's Role
Quantum annealing is a specific type of quantum computing particularly well-suited for optimization problems—problems abundant in drug discovery. Unlike universal quantum computers, quantum annealers are designed to solve optimization problems exceptionally efficiently. This is achieved by exploiting the principles of quantum mechanics to explore a vast solution space and find the global optimum much faster than classical algorithms.
- Finding optimal configurations of molecules for desired properties: Quantum annealing helps find the most stable and effective molecular configurations for a drug candidate.
- Identifying the most promising drug candidates from vast chemical spaces: It allows researchers to sift through enormous databases of potential drug molecules and quickly pinpoint the most promising candidates.
- Optimizing drug delivery methods: Quantum annealing can optimize drug delivery systems, for example, designing nanoparticles for targeted drug delivery with improved efficiency and reduced side effects.
The Synergy of AI and Quantum Computing
The power of quantum computing in drug discovery is further amplified by its integration with artificial intelligence (AI), particularly machine learning. AI excels at analyzing large datasets, identifying patterns, and making predictions – all essential tasks in drug discovery.
AI-Driven Drug Discovery
Machine learning algorithms are increasingly used in drug development to:
- Analyze large datasets of biological information: AI can process and interpret massive datasets of genomic, proteomic, and clinical data to identify potential drug targets and biomarkers.
- Identify patterns and correlations to predict drug efficacy: By analyzing historical drug development data, AI can predict the likelihood of success for new drug candidates.
- Design novel drug molecules based on learned patterns: AI can generate novel drug molecule designs based on learned relationships between molecular structure and biological activity.
Integrating AI with D-Wave's Quantum System
D-Wave's quantum annealers can significantly enhance AI algorithms used in drug discovery by providing:
- Faster training of machine learning models: Quantum computing can accelerate the training process of machine learning models, allowing for faster model development and improved accuracy.
- More accurate predictions of drug properties: Integrating quantum simulations into AI models can lead to more accurate predictions of drug efficacy, toxicity, and other key properties.
- Improved optimization of AI-driven drug design workflows: Quantum annealing can optimize the AI-driven drug design process itself, leading to more efficient and effective drug discovery.
Real-World Applications and Case Studies
While many applications are still in the research phase, the potential of D-Wave's technology is significant. (Note: Specific examples of collaborations and demonstrated improvements would be included here if available from D-Wave's public resources or publications. This section would be significantly expanded with concrete examples to enhance SEO and reader engagement.)
Collaboration with Pharmaceutical Companies
(Insert specific examples of partnerships and collaborations here, citing sources.)
Demonstrated Improvements in Speed and Efficiency
(Insert data and results demonstrating the speed and efficiency gains achieved using D-Wave's technology compared to traditional methods. Cite sources and include quantifiable results where possible.)
Conclusion
D-Wave's quantum computing technology, particularly when integrated with AI, represents a significant leap forward in accelerated drug discovery. The ability to rapidly simulate molecular interactions, optimize drug structures, and enhance AI-driven predictions has the potential to drastically reduce the time and cost associated with bringing life-saving medications to market. This convergence of quantum computing and AI is not just incremental improvement; it's a paradigm shift with the power to transform the pharmaceutical industry.
Call to Action: Learn more about how D-Wave's (QBTS) quantum computing and AI integration are revolutionizing accelerated drug discovery and shaping the future of pharmaceutical research. Explore the potential of quantum computing to transform your drug development process.
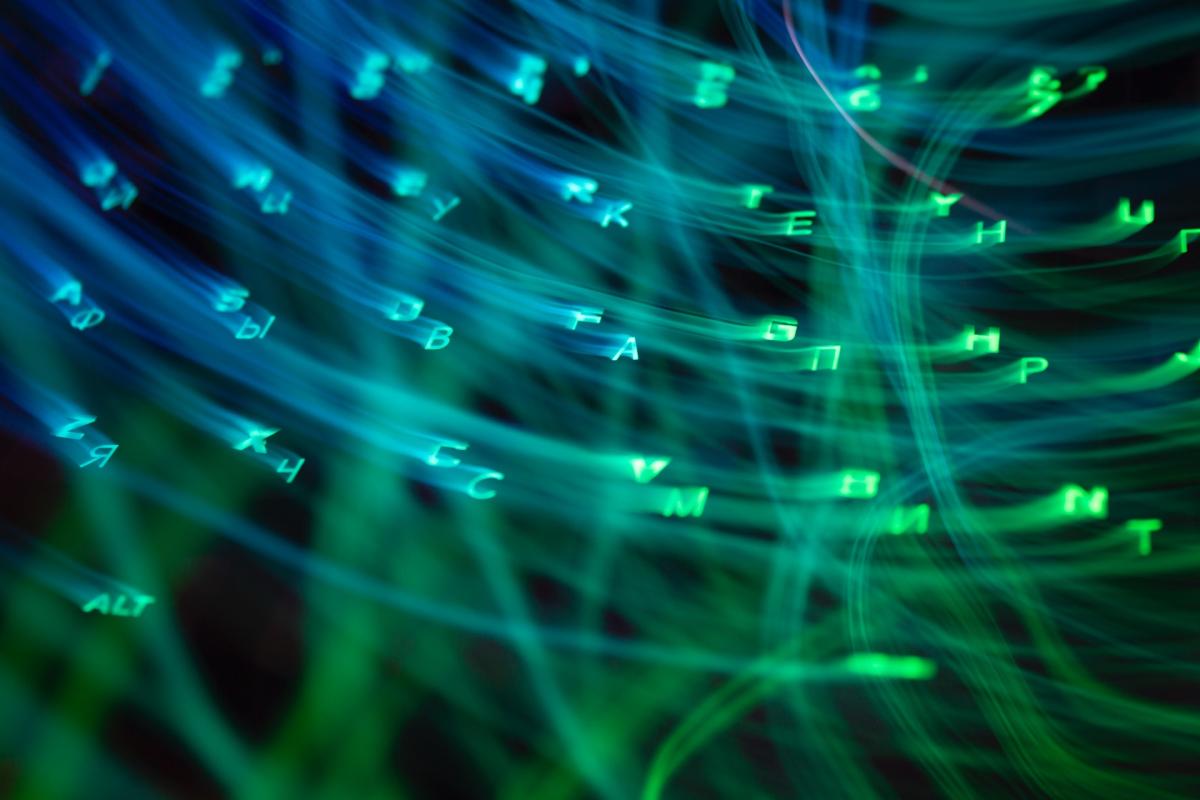
Featured Posts
-
Kaellman Ja Hoskonen Puolalaisura Paeaettynyt
May 20, 2025 -
Druzya Rasskazyvayut O Slozhnoy Situatsii S Mikhaelem Shumakherom
May 20, 2025 -
Affaire Aramburu Point Sur L Enquete Et La Recherche Des Fugitifs D Extreme Droite
May 20, 2025 -
The Ultimate Guide To Solo Travel Planning
May 20, 2025 -
Cours D Ecriture Inspires D Agatha Christie Par L Ia Analyse D Une Innovation
May 20, 2025
Latest Posts
-
Rhea Ripley And Roxanne Perez 2025 Money In The Bank Ladder Match Qualifiers
May 20, 2025 -
Avauskokoonpano Julkistettu Kamara Ja Pukki Vaihtopenkillae
May 20, 2025 -
Jalkapallo Friisin Valinnat Kamara Ja Pukki Penkillae
May 20, 2025 -
Friisin Yllaettaevae Avauskokoonpano Kamara Ja Pukki Sivussa
May 20, 2025 -
Huuhkajien Avauskokoonpano Kaellman Ja Kaksi Muuta Vaihtopenkille
May 20, 2025