AI-Powered Drug Discovery: D-Wave's (QBTS) Quantum Computing Approach
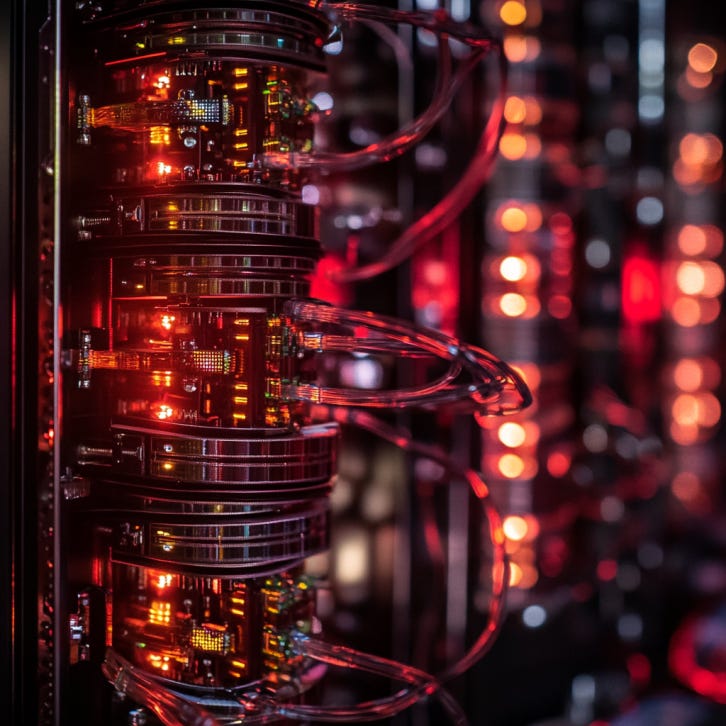
Table of Contents
D-Wave's Quantum Annealing Technology and its Application to Drug Discovery
D-Wave's quantum annealing technology offers a unique approach to solving complex optimization problems, significantly outperforming classical computing methods in certain areas. Unlike classical computers that explore solutions sequentially, quantum annealing leverages the principles of quantum mechanics to explore many solutions simultaneously, greatly accelerating the search for optimal outcomes. This is particularly advantageous in drug discovery, where researchers face the challenge of sifting through vast chemical spaces to identify potential drug candidates.
- Superior performance in solving optimization problems relevant to drug discovery: Quantum annealing excels at finding the global minimum of complex energy landscapes, crucial for tasks like molecular docking and protein folding prediction, both essential for drug development.
- Faster exploration of vast chemical spaces to identify potential drug candidates: The parallel processing power of quantum annealing allows for a much faster screening of potential drug molecules compared to traditional methods, significantly reducing development timelines.
- Potential for identifying novel drug targets and mechanisms of action: By analyzing complex biological data with unprecedented speed, quantum annealing can uncover previously unknown drug targets and potential mechanisms of action, leading to the development of innovative therapeutics.
- Enhanced accuracy in predicting drug efficacy and toxicity: Improved accuracy in predicting these crucial properties can reduce the risk of failure in clinical trials, saving time and resources.
AI Algorithms Integrated with D-Wave's Quantum Computer for Drug Discovery
D-Wave's quantum computers are not used in isolation; their power is amplified through integration with advanced AI algorithms. Machine learning and deep learning models are trained on massive datasets of molecular structures, biological information, and drug properties. These models, then, utilize the quantum computer's capabilities to optimize the search for promising drug candidates.
- Examples of specific AI algorithms used: Algorithms such as support vector machines (SVMs), neural networks, and genetic algorithms are employed, leveraging the quantum computer's ability to handle complex, high-dimensional data.
- How these algorithms leverage the quantum computer's capabilities for enhanced performance: The quantum computer's ability to perform highly parallelized computations allows these algorithms to process vast amounts of data much faster and more efficiently than classical computers.
- Explanation of the data sets used to train and validate these algorithms: Large-scale datasets from sources such as public databases (e.g., PubChem), research publications, and internal pharmaceutical company resources are utilized.
- Discussion on the scalability of the AI-quantum hybrid approach: While still under development, this hybrid approach shows significant promise for scaling to even more complex problems in drug discovery.
Predicting Molecular Properties and Interactions
Accurately predicting molecular properties is critical for successful drug design. Quantum computing dramatically accelerates and enhances the accuracy of these predictions.
- Examples of molecular properties: Binding affinity (how strongly a drug binds to its target), solubility (how well a drug dissolves in the body), stability (how long a drug remains effective), and ADMET properties (absorption, distribution, metabolism, excretion, and toxicity).
- How D-Wave's technology improves the accuracy and speed of these predictions: Quantum annealing's ability to efficiently navigate complex energy landscapes leads to more accurate predictions of these crucial properties, resulting in better drug candidates from the outset.
- Impact on reducing the time and cost of preclinical drug development: By improving the accuracy of predictions, the need for expensive and time-consuming experimental validation is reduced, streamlining the preclinical development process.
Identifying Potential Drug Targets
Identifying promising drug targets is a significant bottleneck in drug discovery. Quantum computing offers a powerful tool to accelerate this process.
- How quantum computing helps analyze vast biological datasets to identify promising targets: Quantum algorithms can rapidly analyze massive datasets of genomic, proteomic, and other biological data to identify potential targets with high accuracy.
- Advantages over traditional methods in terms of speed and efficiency: The speed and efficiency gains are substantial, allowing researchers to explore a much broader range of potential targets in a shorter timeframe.
- Potential for discovering targets previously inaccessible with classical methods: Quantum computing may uncover novel drug targets that would be impossible to identify using classical computational methods.
Case Studies and Real-World Applications of D-Wave's Technology in Drug Discovery
While specific details of ongoing projects are often confidential due to competitive reasons, D-Wave has actively engaged in collaborations with pharmaceutical companies and research institutions, exploring the use of quantum annealing for drug discovery. These collaborations have yielded promising results, although widely publicized case studies are still emerging in this relatively new field. Future publications are expected to highlight significant successes.
Future Outlook and Challenges in AI-Powered Drug Discovery using Quantum Computing
The future of AI-powered drug discovery using quantum computing holds immense potential. However, significant challenges remain.
- Potential breakthroughs and advancements in the near future: We can expect to see increasingly sophisticated AI-quantum hybrid algorithms and more widespread adoption of quantum computing in pharmaceutical research.
- Limitations and challenges currently faced: Computational cost remains a significant barrier, along with the need for further development of efficient quantum algorithms specifically tailored for drug discovery applications.
- Areas for future research and development: Focus areas include developing more powerful quantum algorithms, improving the scalability of the hybrid approach, and generating even larger, more comprehensive datasets for training AI models.
Conclusion:
D-Wave's (QBTS) quantum computing approach is revolutionizing AI-powered drug discovery. By leveraging the power of quantum annealing and integrating it with sophisticated AI algorithms, D-Wave is accelerating the identification and development of new drugs. While challenges remain, the potential for faster, more efficient, and more effective drug development through this technology is undeniable. To stay at the forefront of this exciting field and learn more about the transformative power of AI-powered drug discovery and D-Wave's innovations, continue exploring their resources and research publications. Embrace the future of medicine with advancements in AI-powered drug discovery.
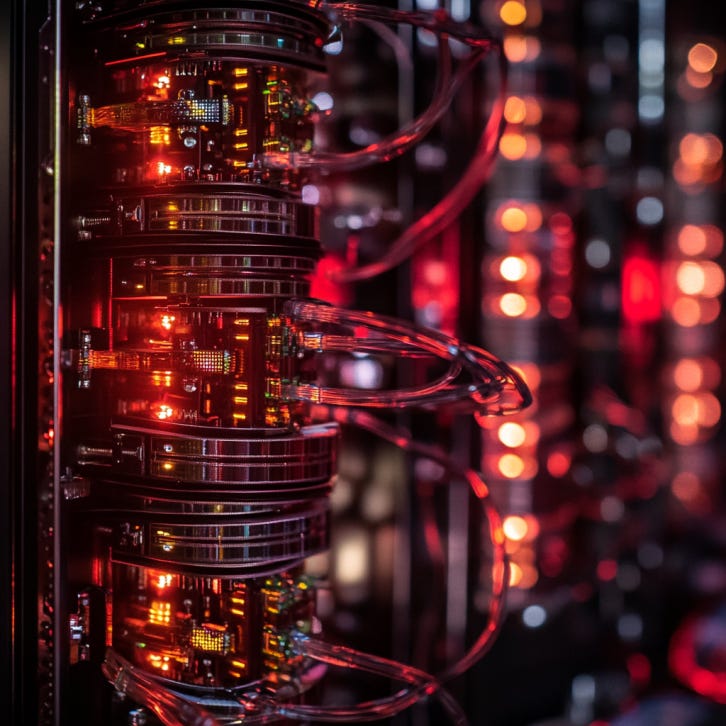
Featured Posts
-
Actor Mark Rylance Speaks Out Against London Music Festivals Impact
May 20, 2025 -
Mark Rylance London Music Festivals Are Like Prison Camps
May 20, 2025 -
Snl Season 50 Finale Shatters Viewership Records
May 20, 2025 -
April 13th Nyt Mini Crossword Complete Answers
May 20, 2025 -
Llm Siri Apples Path To Ai Dominance
May 20, 2025
Latest Posts
-
Roxanne Perez And Rhea Ripley Qualify For The 2025 Money In The Bank Ladder Match
May 20, 2025 -
Huuhkajat Avauskokoonpanoon Kolme Muutosta Kaellman Penkille
May 20, 2025 -
2025 Money In The Bank Perez And Ripley Earn Their Place In The Ladder Match
May 20, 2025 -
Rhea Ripley And Roxanne Perez 2025 Money In The Bank Ladder Match Qualifiers
May 20, 2025 -
Avauskokoonpano Julkistettu Kamara Ja Pukki Vaihtopenkillae
May 20, 2025