AI's Cognitive Limits: Understanding How AI Processes Information
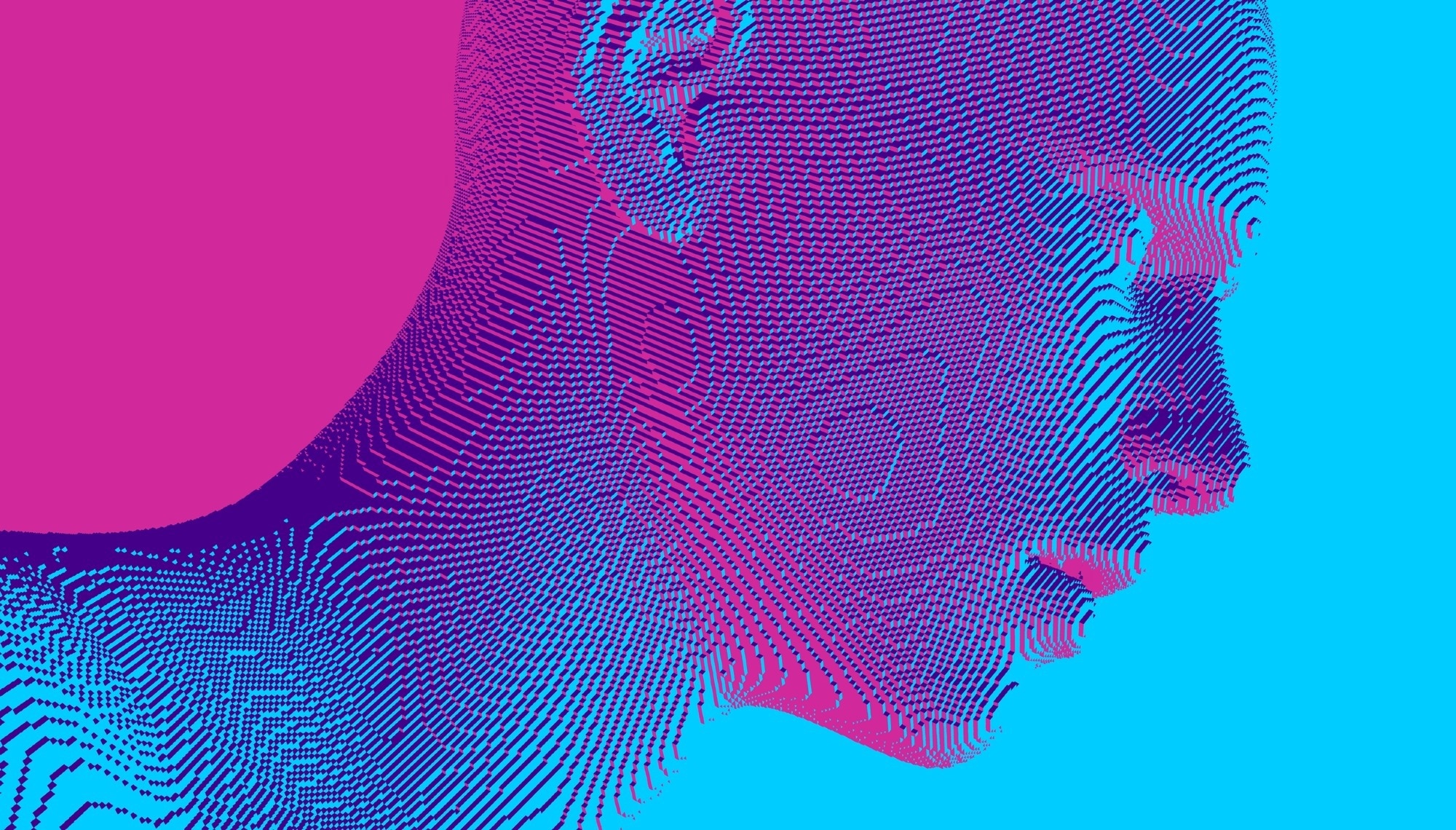
Table of Contents
The Limitations of Symbolic Reasoning in AI
AI often struggles with the nuanced understanding of context and ambiguity that comes naturally to humans. This limitation stems from the predominantly symbolic reasoning approach employed in many AI systems. While effective for structured tasks, symbolic AI falls short when faced with the complexities of real-world scenarios.
-
Difficulty with common sense reasoning and real-world knowledge application: AI systems often lack the broad, intuitive understanding of the world that humans possess. They struggle to apply common sense reasoning to solve problems or make decisions in unfamiliar situations. This is because common sense isn't easily codified into rules and algorithms.
-
Challenges in handling unexpected situations or deviations from programmed scenarios: AI systems are typically trained on specific datasets and operate within predefined parameters. When faced with unexpected situations or deviations from these parameters, their performance can degrade significantly. Robustness and adaptability remain significant challenges.
-
Dependence on structured data and pre-defined rules, limiting adaptability: Symbolic AI relies heavily on structured data and explicitly programmed rules. This dependence limits its ability to adapt to new situations or learn from unstructured data, unlike human cognition which is far more flexible.
-
Example: AI systems often struggle to interpret sarcasm or metaphors, which rely heavily on contextual understanding and implicit meaning. A human easily grasps the irony in a statement like "Oh, fantastic," when used to express displeasure, but an AI may misinterpret the literal meaning.
Keywords: Symbolic AI, Knowledge Representation, Contextual Understanding, Ambiguity Resolution
Data Dependency and the Bias Problem in AI
The performance of AI systems is intrinsically linked to the data they are trained on. This data dependency creates a significant vulnerability: biased or incomplete data leads to flawed, and sometimes discriminatory, outcomes. This is a critical aspect of understanding AI's cognitive limits.
-
AI algorithms are trained on data; biased data leads to biased AI: If the training data reflects existing societal biases, the resulting AI system will likely perpetuate and even amplify those biases. This is a major concern across various applications.
-
The importance of diverse and representative datasets: Creating fair and unbiased AI requires careful curation of training data to ensure it is representative of the diverse populations and contexts the AI will interact with. This is a crucial step in mitigating bias.
-
The challenge of identifying and mitigating bias in training data: Identifying and eliminating bias in data is a complex and ongoing challenge. Techniques like data augmentation and algorithmic fairness interventions are being explored, but this remains an active area of research.
-
Example: Facial recognition systems have demonstrated bias against certain ethnic groups, reflecting biases present in the datasets used to train these systems. This highlights the critical need for careful data selection and bias mitigation strategies.
Keywords: Data Bias, Algorithmic Bias, Machine Learning Bias, Fairness in AI
The Lack of Generalization and Transfer Learning in AI
A key difference between human and artificial cognition is the ability to generalize knowledge. Humans readily transfer knowledge and skills learned in one context to new, seemingly unrelated situations. AI, particularly Narrow AI, struggles with this crucial aspect of intelligence.
-
Narrow AI excels in specific tasks but struggles with broader applications: Current AI systems are typically designed to excel in specific, narrowly defined tasks. They struggle to generalize their knowledge to broader applications or adapt to different contexts.
-
The challenge of transferring learned skills to new, unrelated domains: Transfer learning, the ability to apply knowledge gained from one task to another, is a major challenge for AI. Humans effortlessly transfer skills; AI often requires retraining for each new task.
-
The need for specialized training data for each new task: This lack of generalization necessitates the creation of large, specialized datasets for each new task, hindering the efficiency and scalability of AI development.
-
Example: An AI trained to identify cats with high accuracy may fail miserably at identifying dogs, even though both are animals with similar visual characteristics. This illustrates the limitations of current AI in generalizing knowledge.
Keywords: General Artificial Intelligence (AGI), Transfer Learning, Domain Adaptation, Narrow AI
Explainability and the "Black Box" Problem in AI
Many advanced AI models, particularly deep learning systems, function as "black boxes." This means that it's difficult to understand the internal processes and reasoning that lead to their outputs. This lack of explainability poses significant challenges for trust, accountability, and responsible AI development.
-
The challenge of interpreting the decision-making process of deep learning models: The complex, multi-layered architectures of deep learning models make it difficult to trace how they arrive at their conclusions. This opacity makes it hard to debug errors or ensure fairness.
-
The importance of explainable AI (XAI) for trust and accountability: Explainable AI (XAI) aims to develop techniques that make the decision-making processes of AI models more transparent and understandable. This is crucial for building trust and ensuring accountability.
-
The implications of "black box" AI for various applications (e.g., healthcare, finance): The lack of explainability in AI is particularly concerning in high-stakes applications like healthcare and finance, where understanding the reasoning behind AI-driven decisions is vital.
-
Example: If an AI system rejects a loan application, it’s crucial to understand why. Without explainability, the applicant is left in the dark, hindering trust and potentially leading to unfair outcomes.
Keywords: Explainable AI (XAI), Transparency in AI, Interpretable AI, Deep Learning Explainability
Conclusion
AI's cognitive limits are not insurmountable, but understanding them is crucial for responsible development and deployment. While AI excels in specific tasks through efficient data processing, its reliance on data, limitations in symbolic reasoning and generalization, and the "black box" problem present significant challenges. By acknowledging these limitations and focusing on creating more robust, explainable, and unbiased AI systems, we can harness the true potential of this transformative technology. Continue exploring the complexities of AI's cognitive limits and contribute to building a more responsible and ethical AI future.
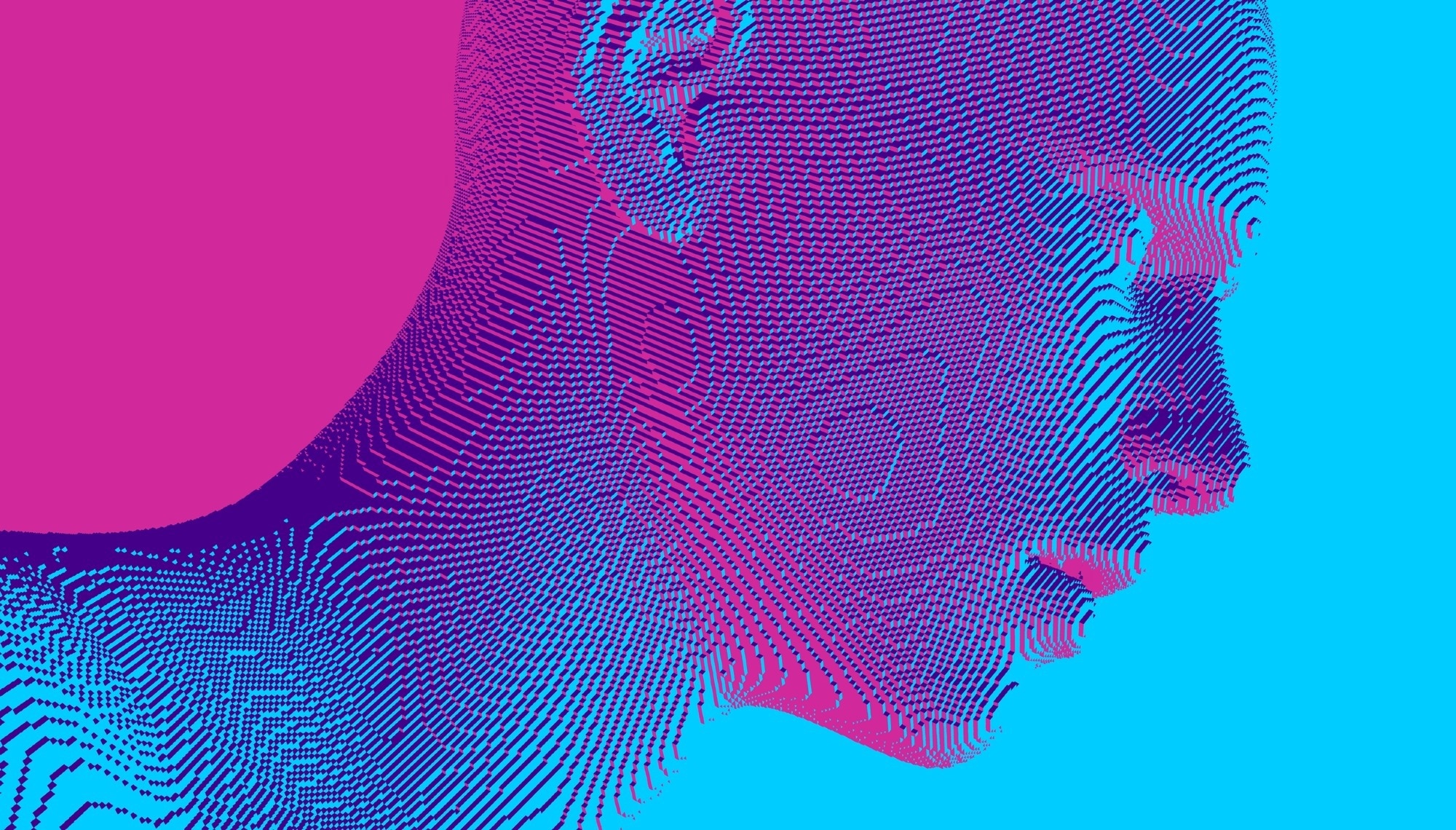
Featured Posts
-
Lab Owner Admits To Falsifying Covid 19 Test Results
Apr 29, 2025 -
River Road Construction Louisville Restaurants Seek Relief
Apr 29, 2025 -
Your Open Thread February 16 2025
Apr 29, 2025 -
February 11th Snow Fox Update Service Impacts And Announcements
Apr 29, 2025 -
Exclusive Universities A Collective Resistance To Trumps Policies
Apr 29, 2025
Latest Posts
-
Tremor On Netflix Release Date Plot And Cast Updates
Apr 29, 2025 -
Pete Rose Pardon Trumps Plans And The Implications For Mlb
Apr 29, 2025 -
Will Netflix Release A Tremor Series What We Know So Far
Apr 29, 2025 -
Will Trump Pardon Pete Rose Examining The Baseball Legends Betting Ban
Apr 29, 2025 -
Is A Tremor Series Coming To Netflix Everything We Know
Apr 29, 2025