Google Vs. OpenAI: A Deep Dive Into I/O And Io
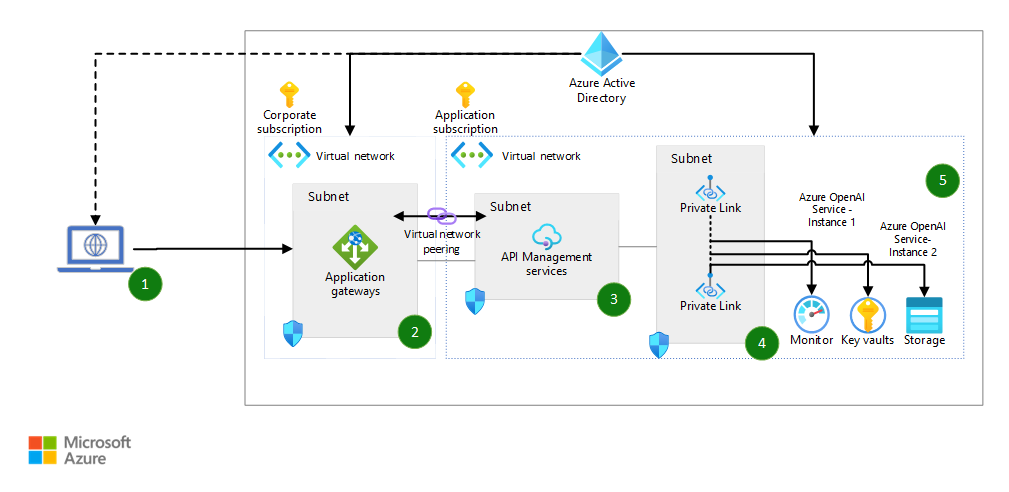
Table of Contents
Google's I/O and io Ecosystem
Google's approach to I/O is deeply intertwined with its vast cloud infrastructure and its commitment to open-source tools. This creates a powerful ecosystem for developers and researchers alike.
Google's Cloud Platform (GCP) and I/O
Google Cloud Platform (GCP) provides a robust and scalable foundation for managing I/O operations. Its comprehensive suite of services simplifies the complexities of data handling, making it ideal for various AI applications.
- Integration with other Google services: Seamless integration with services like Google Drive, BigQuery, and other Google Cloud services allows for streamlined data pipelines and efficient I/O management. This interconnectedness reduces latency and improves overall workflow efficiency.
- Strong emphasis on serverless computing and managed services: GCP's serverless offerings, like Cloud Functions and Cloud Run, abstract away the complexities of server management, allowing developers to focus on I/O logic and application development. This simplification improves development speed and reduces operational overhead.
- Advanced tools for data processing and machine learning I/O pipelines: Tools like Dataflow, Dataproc, and Vertex AI provide powerful capabilities for processing large datasets and building efficient machine learning I/O pipelines. This ensures smooth data flow and optimized performance for demanding AI applications.
- Examples of successful I/O implementations using GCP: Numerous successful applications demonstrate the power of GCP's I/O capabilities. From large-scale data analytics projects to real-time machine learning deployments, GCP's flexibility and scalability have proven invaluable.
Google's Approach to Natural Language Processing (NLP) I/O
Google is a leader in Natural Language Processing (NLP), and its I/O methodologies reflect this expertise. Models like BERT, LaMDA, and PaLM showcase Google's commitment to contextual understanding and sophisticated I/O interactions.
- Focus on contextual understanding and sophisticated I/O interactions: Google's NLP models excel at understanding the nuances of language, allowing for more accurate and contextually relevant I/O. This translates to more natural and human-like interactions with AI systems.
- Comparison of Google's NLP I/O to other platforms: Compared to other platforms, Google's NLP I/O often demonstrates superior performance in tasks such as question answering, text summarization, and machine translation due to its massive training datasets and advanced model architectures.
- Real-world applications demonstrating Google's NLP I/O capabilities: Google Assistant, Google Translate, and Search are prime examples of how Google's advanced NLP I/O is used to enhance user experiences in real-world applications.
- Limitations and future directions for Google's NLP I/O: While Google's NLP I/O is highly advanced, ongoing research focuses on improving efficiency, reducing bias, and enhancing the capabilities of its models for even more natural and intuitive interactions.
OpenAI's I/O and io Architecture
OpenAI's approach to I/O is markedly different from Google's, emphasizing API accessibility and cutting-edge model development. This approach prioritizes ease of use and rapid innovation.
OpenAI's API and I/O
OpenAI's API-centric approach makes its powerful models readily accessible to developers. This simplicity fosters rapid prototyping and integration into various applications.
- Simplicity of integration for developers: The straightforward nature of OpenAI's API simplifies the integration process, allowing developers to quickly incorporate advanced AI capabilities into their projects.
- Focus on specific model I/O (e.g., text-to-text, image-to-text): OpenAI's models are often specialized for particular I/O tasks, enabling developers to choose the best tool for their needs. This specialized focus often results in higher accuracy for specific tasks.
- Community contributions and model extensions impacting I/O: OpenAI fosters a vibrant community, leading to ongoing improvements and extensions of its models, constantly enhancing I/O capabilities.
- Limitations related to control and customization of I/O processes: The trade-off for ease of use is sometimes reduced control over the underlying I/O processes. Developers might have less granular control compared to Google Cloud Platform's offerings.
OpenAI's Research Focus and Future I/O
OpenAI's commitment to research drives continuous innovation in its I/O capabilities. Techniques like Reinforcement Learning from Human Feedback (RLHF) are crucial to improving the quality and safety of its models.
- Impact of RLHF on improving I/O accuracy and user experience: RLHF significantly enhances the user experience by aligning AI models with human preferences, resulting in more natural and helpful I/O.
- Exploration of multimodal I/O (combining text, images, audio): OpenAI is actively exploring multimodal I/O, pushing the boundaries of AI interaction beyond text-based input and output. This signifies a significant leap toward more human-like interactions with AI.
- Ethical considerations related to OpenAI's I/O and its potential societal impact: OpenAI actively addresses the ethical implications of its technology, ensuring responsible development and deployment of its I/O capabilities.
- Predictions for future advancements in OpenAI's I/O capabilities: Future advancements are likely to focus on even more advanced multimodal I/O, improved safety and robustness, and increased efficiency.
Google vs. OpenAI: A Comparative Analysis of I/O
Direct comparison reveals distinct strengths and weaknesses in Google and OpenAI's approaches to I/O.
- Comparison of scalability, cost-effectiveness, ease of use, and security: Google emphasizes scalability and a comprehensive ecosystem, while OpenAI prioritizes ease of use and cutting-edge model capabilities. Cost-effectiveness varies depending on specific needs and usage patterns. Security is a paramount concern for both platforms.
- Discussion of specific use cases where one platform excels over the other: Google's platform excels in large-scale data processing and complex I/O workflows, while OpenAI's API is ideal for quick prototyping and integration of cutting-edge models.
- Analysis of the long-term implications of each approach: Both approaches are likely to continue to evolve, shaping the future of AI and its applications.
Conclusion
This deep dive into Google and OpenAI reveals distinct yet equally impactful approaches to I/O and their underlying "io" infrastructure. Google emphasizes a comprehensive, integrated ecosystem with strong scalability and a focus on diverse data processing needs. OpenAI prioritizes ease of access and cutting-edge model development, leading to innovative I/O capabilities, particularly in natural language processing. The choice between Google and OpenAI depends heavily on specific project requirements and priorities. By understanding the nuances of their I/O strategies, developers and users can make informed decisions and leverage the power of these leading AI platforms to build the future of technology. To further explore the possibilities of advanced I/O and "io," consider experimenting with both Google's and OpenAI's offerings to find the best fit for your needs.
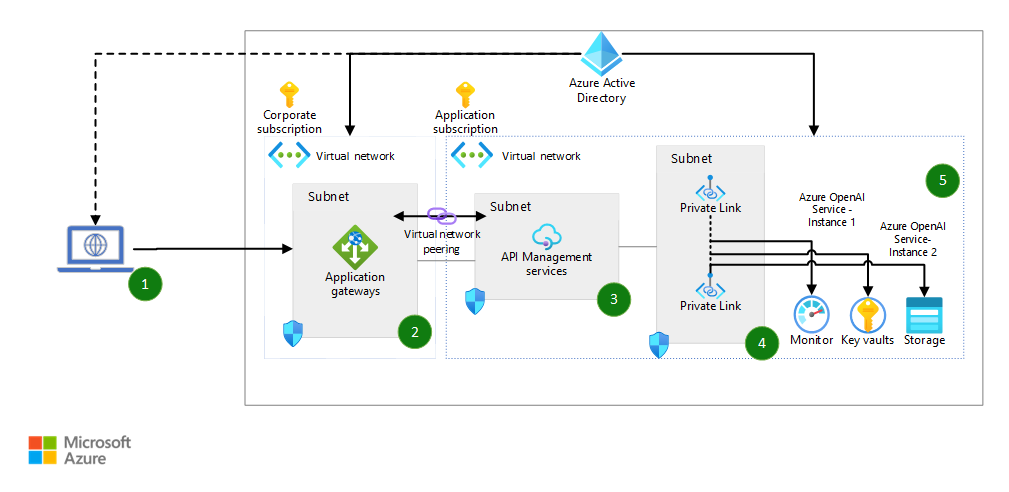
Featured Posts
-
The Railway Station Man Observing The Comings And Goings
May 26, 2025 -
Polsce24 Demaskuje Blamaz Prokuratorow Unikanie Odpowiedzialnosci
May 26, 2025 -
The Rise Of Alternative Delivery Options In Response To Canada Post Issues
May 26, 2025 -
Paris Roubaix Police Apprehend Spectator Who Threw Bottle At Mathieu Van Der Poel
May 26, 2025 -
Thames Water Executive Compensation A Critical Evaluation
May 26, 2025