How Outdated Business Apps Hamper AI Integration
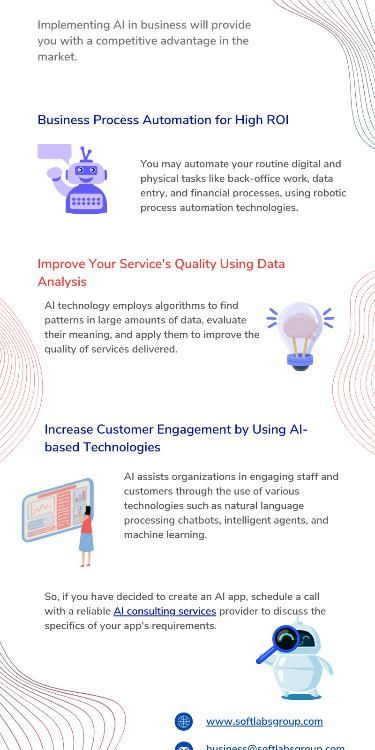
Table of Contents
Data Incompatibility and Accessibility Issues
Outdated business applications present significant hurdles to effective AI integration, primarily through data incompatibility and accessibility problems. Successfully implementing AI requires clean, structured, and readily accessible data – a resource often lacking when dealing with legacy systems.
Legacy Systems Lacking Modern Data Structures
Outdated apps often rely on antiquated data formats (e.g., flat files, proprietary databases) incompatible with modern AI tools. These tools typically require structured, clean data in formats like CSV, JSON, or database systems optimized for querying and analysis.
- Difficulty in extracting, transforming, and loading (ETL) data for AI model training: The process of preparing data for AI models becomes significantly more complex and time-consuming.
- Increased data cleansing and preprocessing costs, delaying AI deployment: Cleaning and transforming incompatible data formats can consume substantial resources, pushing back project timelines and increasing costs.
- Risk of inaccurate AI insights due to poor data quality: Inconsistent, incomplete, or inaccurate data leads to flawed AI models, rendering their insights unreliable and potentially harmful to business decisions.
Limited API Integrations and Data Sharing
Older systems may lack robust Application Programming Interfaces (APIs), hindering seamless integration with AI platforms and other crucial business systems. This limitation creates data silos and hampers efficient data sharing.
- Manual data transfer, leading to errors and delays: The need for manual data transfer increases the risk of human error, slowing down the entire AI integration process.
- Difficulty in real-time data analysis for AI-driven decision-making: Lack of real-time data access prevents the use of AI for timely and effective decision-making.
- Increased risk of data silos and inconsistent data across the organization: Data trapped in incompatible systems leads to fragmented information, hindering a holistic view of the business and reducing the effectiveness of AI analysis.
Integration Challenges and Scalability Limitations
Integrating AI with outdated systems presents substantial integration challenges and scalability limitations, significantly impacting the return on investment (ROI) of AI initiatives.
Complexity of Integrating AI with Legacy Systems
Integrating AI requires significant effort and resources when dealing with outdated, inflexible systems, often necessitating costly custom development. This adds complexity and risk to the project.
- Increased development time and budget for AI projects: Custom integrations are time-consuming and expensive, potentially exceeding initial budget projections.
- Potential for integration failures due to incompatibility issues: The risk of failure increases due to unforeseen compatibility problems between legacy systems and modern AI platforms.
- Higher risk of security vulnerabilities due to outdated security protocols in legacy systems: Older systems might lack robust security measures, creating vulnerabilities that can compromise the entire AI infrastructure.
Scalability Issues and Lack of Flexibility
Older systems often struggle to handle the increased data volume and processing demands associated with AI applications. This limits the scalability and adaptability of AI initiatives.
- Performance bottlenecks and system failures when processing large datasets: Outdated systems may not be able to handle the large datasets often used for training complex AI models.
- Inability to quickly adapt to changing business needs and incorporate new AI technologies: Legacy systems are inflexible and resistant to change, making it difficult to adopt new AI advancements.
- Difficulty in scaling AI solutions across different departments and locations: Scaling AI across the entire organization becomes challenging if the underlying systems are not designed for scalability.
Security Risks and Compliance Concerns
Outdated business applications pose significant security risks and compliance concerns, impacting the responsible and ethical deployment of AI within the organization.
Outdated Security Protocols and Vulnerabilities
Legacy systems may lack essential security features, increasing the risk of data breaches and compromising the integrity of AI models and sensitive business information.
- Higher susceptibility to cyberattacks and data loss: Older systems are more vulnerable to cyberattacks due to outdated security protocols and lack of patching.
- Non-compliance with data privacy regulations (e.g., GDPR, CCPA): Failure to meet data privacy regulations can result in significant financial penalties and reputational damage.
- Reputational damage and financial losses due to security breaches: Data breaches can lead to significant financial losses, legal battles, and reputational harm.
Difficulty in Ensuring AI Model Transparency and Explainability
Outdated systems can obscure the decision-making processes of AI models, making it challenging to ensure transparency, accountability, and compliance with regulatory requirements.
- Difficulty in understanding how AI models arrive at their conclusions: Lack of transparency can hinder the ability to audit and validate AI model decisions.
- Lack of audit trails for AI-driven actions: This lack of accountability can increase the risk of errors and biases going undetected.
- Increased risk of biased or unfair outcomes from AI systems: Without proper monitoring and explainability, AI models can perpetuate existing biases present in the data, leading to unfair or discriminatory outcomes.
Conclusion
Outdated business applications significantly impede successful AI integration by creating data incompatibility, integration complexities, scalability limitations, and security risks. Addressing these challenges is crucial for maximizing the benefits of AI and gaining a competitive edge. Ignoring these issues can lead to wasted resources, missed opportunities, and even reputational damage.
Call to Action: Upgrade your business applications and embrace modern cloud-based solutions to unlock the full potential of AI. Don't let outdated software hamper your AI integration strategy – invest in modernization to drive innovation and growth. Learn more about how to successfully integrate AI by exploring resources on modernizing your business applications and implementing robust data management strategies. Your journey to successful AI integration starts with modernizing your technological foundation.
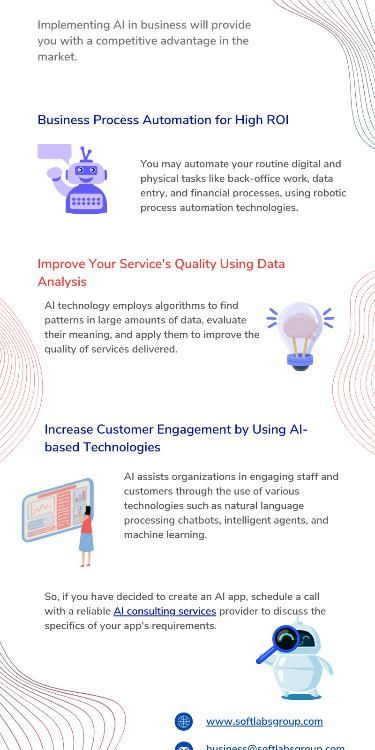
Featured Posts
-
Hasbros New Star Wars Shadow Of The Empire Dash Rendar Figure
May 01, 2025 -
Stroomproblemen Kampen School Start Kort Geding Tegen Enexis
May 01, 2025 -
Cavs Week 16 Takeaways Big Trade Much Needed Break
May 01, 2025 -
Hollywood Actors Rs 8 7 Crore Charity After Tata Steel Layoffs
May 01, 2025 -
Wayne Gretzkys Fast Facts A Quick Look At The Great Ones Career
May 01, 2025
Latest Posts
-
Chris Kaba Panorama Police Watchdog Files Ofcom Complaint
May 01, 2025 -
Panoramas Chris Kaba Episode Iopc Complaint To Ofcom
May 01, 2025 -
Ofcom Under Scrutiny Police Complaint Over Chris Kaba Panorama
May 01, 2025 -
26 2025 12
May 01, 2025 -
Chris Kaba Panorama Police Watchdog Challenges Bbc Coverage With Ofcom Complaint
May 01, 2025