Improving The Accuracy Of Automated Visual Inspection For Lyophilized Vials
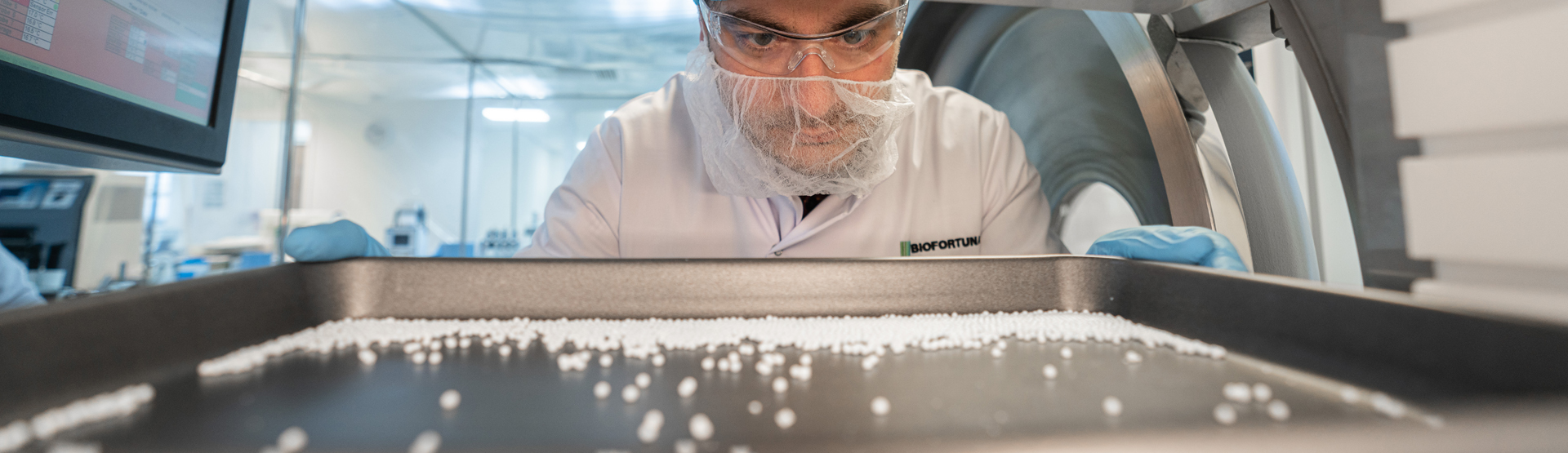
Table of Contents
Challenges in Automated Visual Inspection of Lyophilized Vials
The inherent variability in lyophilization processes and the complexities of image analysis contribute significantly to the difficulties in achieving high accuracy with AVI for lyophilized vials.
Variability in Lyophilization Processes
The lyophilization process itself introduces variability in the final product's appearance. This variability directly impacts the effectiveness of automated visual inspection systems.
- Variations in cake appearance: Cracks, collapse, inconsistencies in the cake's structure, and variations in its color are common. These variations can be subtle and difficult for automated systems to reliably detect.
- Differences in fill levels: Inconsistent fill levels within vials can lead to misinterpretations by the inspection system, potentially flagging acceptable vials as defective.
- Presence of particulate matter: The presence of small particles within the vial or on the stopper can be challenging to detect, especially when using lower-resolution imaging systems.
These variations make it difficult for image processing algorithms to establish consistent thresholds for identifying defects, resulting in a higher rate of false positives (rejecting good vials) or false negatives (accepting defective vials). The inconsistency necessitates sophisticated algorithms capable of adapting to a wide range of visual presentations.
Lighting and Shadowing Effects
Variations in lighting and the presence of shadows within the vials significantly affect image analysis. Poor lighting conditions can obscure defects or create artifacts that the system interprets as defects.
- Uneven illumination: Inconsistent illumination across the vial can lead to variations in image brightness, making defect detection unreliable.
- Reflections: Reflections from the vial's surface or the lyophilized cake can mask or mimic defects.
- Shadows obscuring defects: Shadows cast by the vial's stopper or the cake itself can hide critical defects from the automated system.
To mitigate these issues, advanced lighting strategies, such as using diffused lighting or multiple light sources, are crucial. Sophisticated image processing techniques that compensate for shadowing and reflections are also necessary for achieving reliable results.
Image Resolution and Algorithm Limitations
Current image processing algorithms and the resolution of imaging systems often limit the ability to detect subtle defects. High-resolution images are required to capture fine details, but this increases the computational cost and processing time.
- Difficulties in detecting subtle defects: Microscopic cracks, small particulate matter, or minor variations in cake structure can be missed by systems with lower resolution or less sophisticated algorithms.
- Limitations of current AI/ML algorithms: While AI and machine learning (ML) algorithms are increasingly used in AVI, their accuracy depends heavily on the quality and quantity of training data. Insufficient or biased data can lead to poor performance.
- Computational cost of high-resolution image processing: Processing high-resolution images requires significant computational power, potentially slowing down the inspection process.
The selection of appropriate image processing techniques, including the optimization of machine learning models specifically for lyophilized vial inspection, is paramount. Exploring the use of deep learning models trained on diverse and representative datasets is crucial for improved accuracy.
Strategies for Enhancing Inspection Accuracy
Several strategies can be implemented to significantly enhance the accuracy of automated visual inspection for lyophilized vials.
Advanced Image Processing Techniques
Employing advanced image processing techniques is key to improving defect detection.
- Deep learning algorithms: Deep learning models, such as convolutional neural networks (CNNs), have shown remarkable success in image recognition tasks and are well-suited for automated visual inspection. They can be trained to identify subtle defects that might be missed by traditional methods.
- Image enhancement techniques: Techniques like noise reduction, contrast adjustment, and sharpening can improve the quality of the images, making defect detection more reliable.
- 3D imaging for improved defect visualization: 3D imaging can provide a more complete view of the vial and its contents, allowing for the detection of defects that might be obscured in 2D images.
Companies specializing in machine vision and AI are actively developing and refining these algorithms for applications in pharmaceutical quality control. Collaboration with these companies and ongoing research into algorithm optimization are crucial for improving accuracy.
Improved Lighting and Calibration
Careful control of lighting and regular calibration of the AVI system are essential for minimizing errors.
- Use of specialized lighting: Utilizing diffused, consistent, and controlled lighting is crucial to eliminate uneven illumination and shadows.
- Calibration standards: Regular calibration using standardized vials with known defects ensures the system's accuracy is maintained over time.
- Regular system maintenance: Regular maintenance and preventative measures are essential to maintain the system’s optimal performance.
- Quality control checks: Implementing robust quality control checks to verify the system's accuracy is essential to ensure the reliability of the inspection process.
Proper calibration and maintenance are not merely operational tasks, but fundamental to maintaining the integrity of the AVI process and ensuring compliance with regulatory requirements.
Data Augmentation and Training
Improving the training datasets used to train AI-based inspection systems is crucial for enhancing their accuracy and robustness.
- Generating synthetic data: Generating synthetic data can augment real-world datasets, especially for rare or difficult-to-obtain defect types.
- Using diverse datasets: The training data should include a wide variety of vials representing different lyophilization processes, fill levels, and defect types.
- Continuous model retraining: Regularly retraining the AI models with updated datasets helps maintain accuracy and adapt to changes in the lyophilization process.
The quality of the training data directly correlates to the algorithm's ability to generalize and accurately classify defects in real-world scenarios.
Future Trends in Automated Visual Inspection of Lyophilized Vials
The future of automated visual inspection for lyophilized vials is bright, with several promising trends on the horizon.
Integration of AI and Machine Learning
AI and machine learning will play an increasingly important role in enhancing AVI systems.
- Predictive maintenance: AI can be used to predict potential equipment failures, minimizing downtime and ensuring consistent inspection performance.
- Real-time defect classification: Advanced AI algorithms can provide real-time defect classification and analysis, facilitating immediate corrective actions.
- Self-learning algorithms: Self-learning algorithms can adapt to changes in the lyophilization process and automatically adjust inspection parameters to maintain optimal accuracy.
This continuous learning capacity holds the potential to revolutionize lyophilized vial quality control and minimize the risk of human error.
Hyperspectral Imaging and other Advanced Technologies
Emerging technologies such as hyperspectral imaging offer enhanced capabilities for defect detection.
- Non-destructive testing capabilities: Hyperspectral imaging provides detailed spectral information, allowing for the detection of defects that are invisible to traditional imaging techniques.
- Ability to detect subtle defects: This technology can detect subtle variations in the lyophilized cake that might indicate inconsistencies in the lyophilization process.
While these technologies are currently in their early stages of development for this specific application, they offer promising avenues for further improving the accuracy and capabilities of automated visual inspection.
Conclusion
Improving the accuracy of automated visual inspection for lyophilized vials is essential for maintaining product quality and regulatory compliance within the pharmaceutical industry. While challenges exist related to process variability, lighting effects, and algorithm limitations, advancements in image processing techniques, AI, and emerging technologies offer significant opportunities for improvement. By incorporating these advanced techniques and strategies into your automated visual inspection system, you can significantly improve the accuracy and reliability of your lyophilized vial quality control processes. Further research and development in this area will continue to drive advancements and improve the overall efficiency and reliability of pharmaceutical manufacturing. Consider contacting industry experts to explore the best solutions for your specific needs in automated visual inspection.
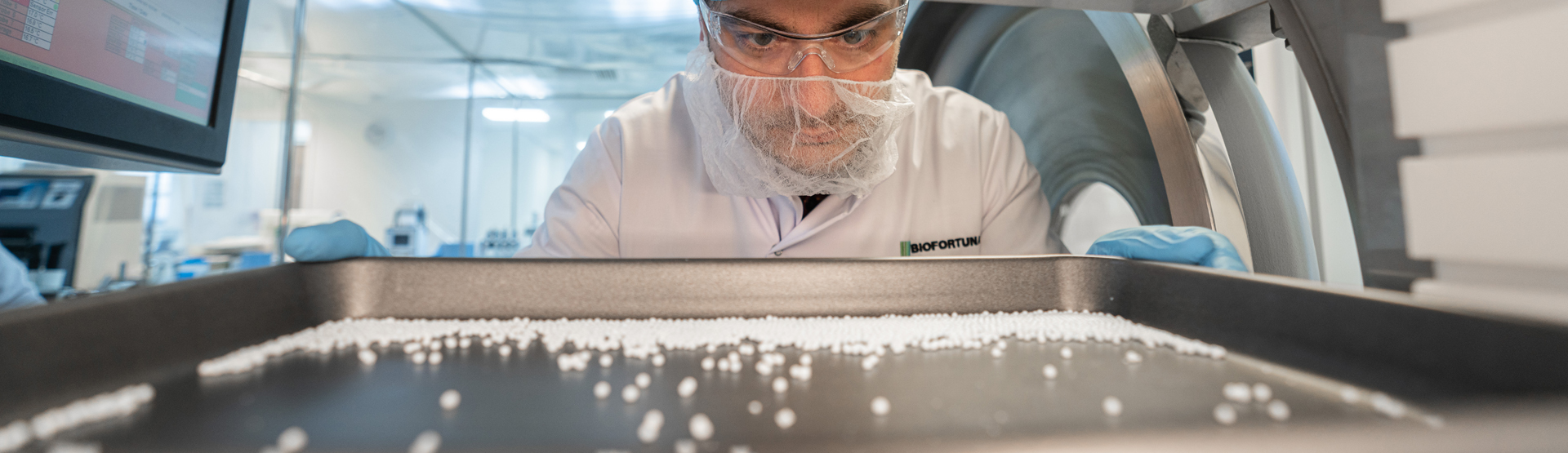
Featured Posts
-
Sir Bradley Wiggins Post Cycling Struggles Addiction Bankruptcy And Recovery
May 12, 2025 -
Exploring Lavish Homes An Mtv Cribs Inspired Tour Of Rich Kids Cribs
May 12, 2025 -
Prince Andrew Accusers Dire Claim Four Days To Live
May 12, 2025 -
Indy 500 2024 34 Car Grid Confirmed With Satos Addition
May 12, 2025 -
Boris Dzhonson Proti Mirnogo Planu Trampa Detalniy Analiz
May 12, 2025
Latest Posts
-
Mtv Movie And Tv Awards Cancelled For 2025 Official Confirmation
May 12, 2025 -
Jessica Simpsons Daughter Birdie 6 Matches Mom In Cute Yellow Swimsuits
May 12, 2025 -
Analyzing The Vma Simulcast On Cbs A Threat To Mtvs Relevance
May 12, 2025 -
The Impact Of Cbss Vma Simulcast On Mtvs Viewership
May 12, 2025 -
Airport Fashion Jessica Simpsons Cheetah Print And Blue Fur Ensemble
May 12, 2025