The Impact Of Outdated Business Applications On AI Implementation
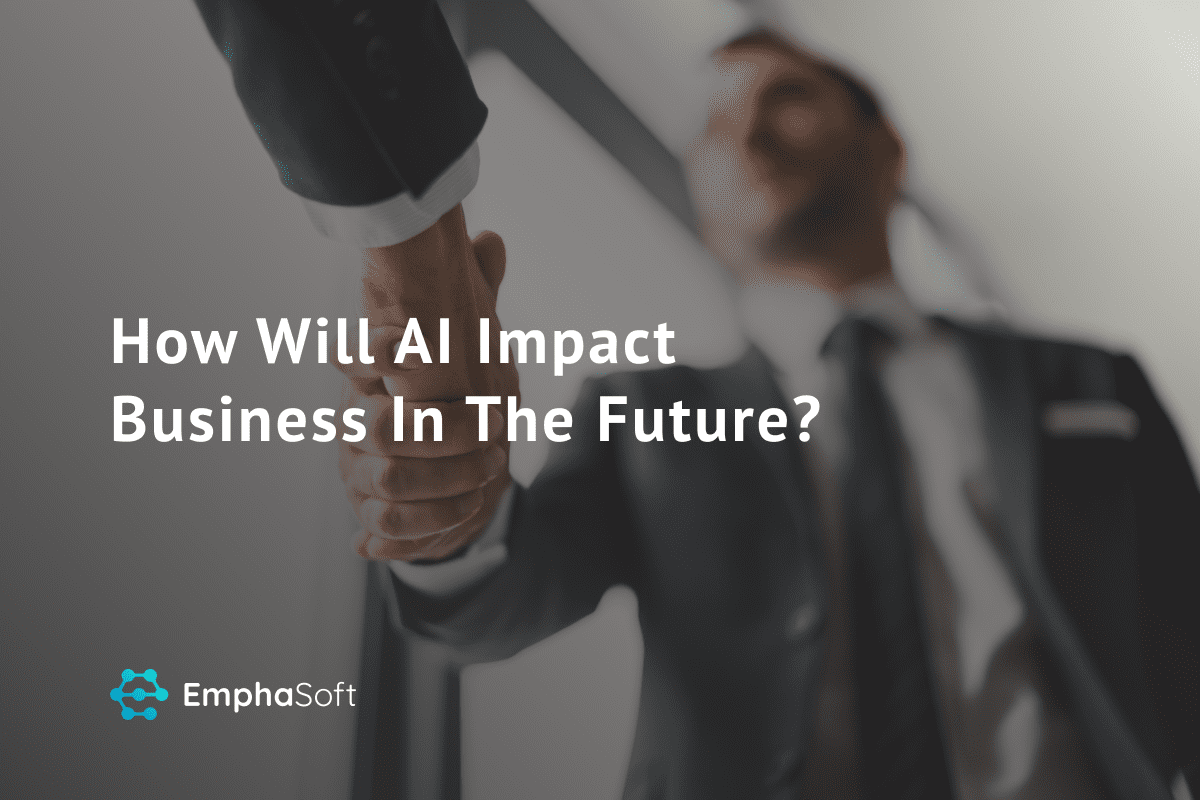
Table of Contents
Data Incompatibility and Integration Challenges
Outdated systems often present significant hurdles when integrating with AI. One of the most pressing issues is data incompatibility. Legacy systems frequently lack robust APIs (Application Programming Interfaces) or utilize incompatible data formats (e.g., proprietary formats, outdated databases), making data integration with modern AI systems a complex and expensive undertaking. This incompatibility leads to several critical problems:
- Difficulty in extracting relevant data for AI model training: AI models require large, clean, and structured datasets for effective training. Extracting this data from disparate, outdated systems can be incredibly time-consuming and error-prone.
- High costs associated with data migration and transformation: Moving data from legacy systems to AI-compatible formats requires significant investment in data migration tools, expertise, and time.
- Increased risk of data errors and inconsistencies: Data cleansing and transformation processes are complex and prone to errors, leading to inaccurate AI models and unreliable results.
- Slower AI model development and deployment timelines: The challenges in data integration directly impact the speed of AI model development and deployment, delaying the realization of ROI.
These data integration challenges associated with legacy systems can significantly slow down or even prevent successful AI implementation. Addressing data compatibility issues is paramount for achieving a smooth and efficient AI integration process.
Security Risks and Compliance Issues
Integrating AI systems with outdated applications introduces significant security risks and compliance concerns. Legacy systems often lack the robust security features necessary to protect sensitive data, especially considering AI often requires access to large volumes of confidential information. This poses several critical threats:
- Increased risk of data breaches and cyberattacks: Outdated systems are more vulnerable to cyberattacks, potentially leading to significant data breaches and financial losses.
- Non-compliance with industry regulations and standards: Failing to meet regulatory requirements like GDPR (General Data Protection Regulation) or HIPAA (Health Insurance Portability and Accountability Act) can result in hefty fines and reputational damage.
- Potential for hefty fines and reputational damage: Data breaches and non-compliance can severely damage a company's reputation and lead to significant financial penalties.
- Difficulty in auditing and monitoring AI systems: Integrating AI with insecure legacy systems makes auditing and monitoring significantly more challenging, hindering effective risk management.
Organizations must prioritize robust security measures when integrating AI with existing systems, especially legacy systems. Regular security assessments and updates are crucial to mitigate these risks.
Scalability and Performance Limitations
Another significant challenge posed by outdated business applications is their inherent limitations in scalability and performance. AI applications, particularly those involving machine learning, often require significant processing power and the ability to handle massive datasets. Legacy systems frequently struggle to meet these demands, resulting in:
- Slower processing speeds and response times: Outdated systems may struggle to process the large volumes of data required for AI applications, resulting in slow response times and impacting user experience.
- Inability to scale to handle larger datasets: As AI models grow and require more data, legacy systems may fail to scale appropriately, hindering the expansion of AI initiatives.
- Increased operational costs due to inefficient resource utilization: The inefficiency of legacy systems can lead to increased operational costs due to resource overuse and underperformance.
- Limited ability to support advanced AI algorithms: Outdated systems may lack the necessary infrastructure to support the computational demands of advanced AI algorithms.
Investing in systems capable of handling the growing data demands of AI is essential for successful implementation.
Lack of Automation and Integration Capabilities
Outdated applications often lack the automation capabilities crucial for seamless integration with AI workflows. This results in manual processes that are inefficient, error-prone, and costly. The consequences include:
- Increased reliance on manual data entry and processing: Manual data entry is time-consuming, prone to errors, and expensive.
- Reduced efficiency and productivity: Manual processes hinder productivity and prevent the efficient execution of AI workflows.
- Higher operational costs due to manual intervention: Manual intervention increases operational costs associated with labor and error correction.
- Increased risk of human error: Manual data handling increases the likelihood of human errors, leading to inaccurate results and compromised data integrity.
Automating data processes is essential for achieving the full benefits of AI integration, and outdated systems often impede this crucial step.
Strategies for Mitigating the Impact
Businesses facing these challenges need to adopt proactive strategies to mitigate the negative impact of outdated applications on AI implementation. Several key approaches can help:
- Gradual modernization of legacy systems: A phased approach to upgrading legacy systems can minimize disruption while incrementally improving compatibility with AI systems.
- Investing in cloud-based solutions: Cloud-based solutions offer scalability, flexibility, and improved security, making them ideal for supporting AI initiatives.
- Implementing data integration platforms: Modern data integration platforms can help bridge the gap between legacy systems and AI platforms, improving data flow and reducing compatibility issues.
- Adopting a phased approach to AI implementation: Implementing AI in stages allows businesses to test and refine their approach, mitigating risks and maximizing efficiency.
- Seeking expert consultation on AI integration: Consulting with AI integration specialists can provide guidance and support throughout the implementation process.
By adopting these strategies, businesses can effectively navigate the challenges posed by outdated applications and successfully integrate AI into their operations.
Conclusion
Outdated business applications present significant challenges to successful AI implementation, including data incompatibility, security risks, scalability limitations, and a lack of automation. These issues can lead to increased costs, delayed timelines, and a reduced return on investment. However, by addressing these issues through strategic modernization, investment in cloud solutions, and careful planning, businesses can overcome these hurdles and unlock the transformative power of AI. Don't let outdated business applications hinder your AI journey. Begin evaluating your systems today and plan a strategic modernization path to unlock the transformative potential of AI and gain a significant competitive advantage. Invest in your future; invest in AI integration.
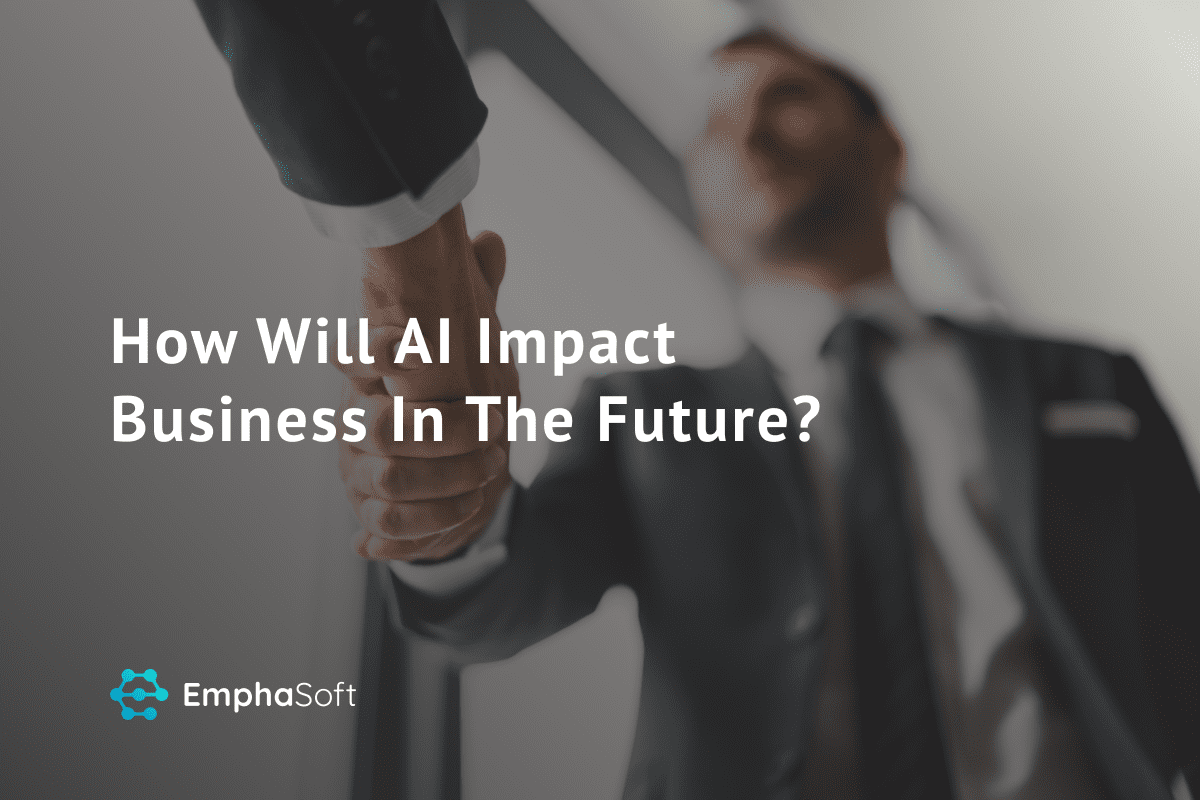
Featured Posts
-
Disney Cruise Line Double The Alaska Fun In Summer 2026
May 01, 2025 -
Legacy Systems And Ai A Recipe For Disaster
May 01, 2025 -
Phipps Challenges Australian Rugbys Supremacy
May 01, 2025 -
Six Nations Roundup France Reigns Supreme England Crushes Wales Scotland And Ireland Struggle
May 01, 2025 -
Xrp Price Surge 400 In 3 Months Is It Time To Buy
May 01, 2025
Latest Posts
-
A Look At Ongoing Nuclear Litigation Key Cases And Trends
May 02, 2025 -
Officieel Bram Endedijk Is De Nieuwe Presentator Van Nrc Vandaag
May 02, 2025 -
Bram Endedijk Presenteert Voortaan Nrc Vandaag
May 02, 2025 -
Bram Endedijk De Nieuwe Presentator Van Nrc Vandaag
May 02, 2025 -
Hundreds Stranded In Kogi Following Train Failure
May 02, 2025