We Now Know How AI "Thinks": A Look At Its Limited Cognitive Abilities
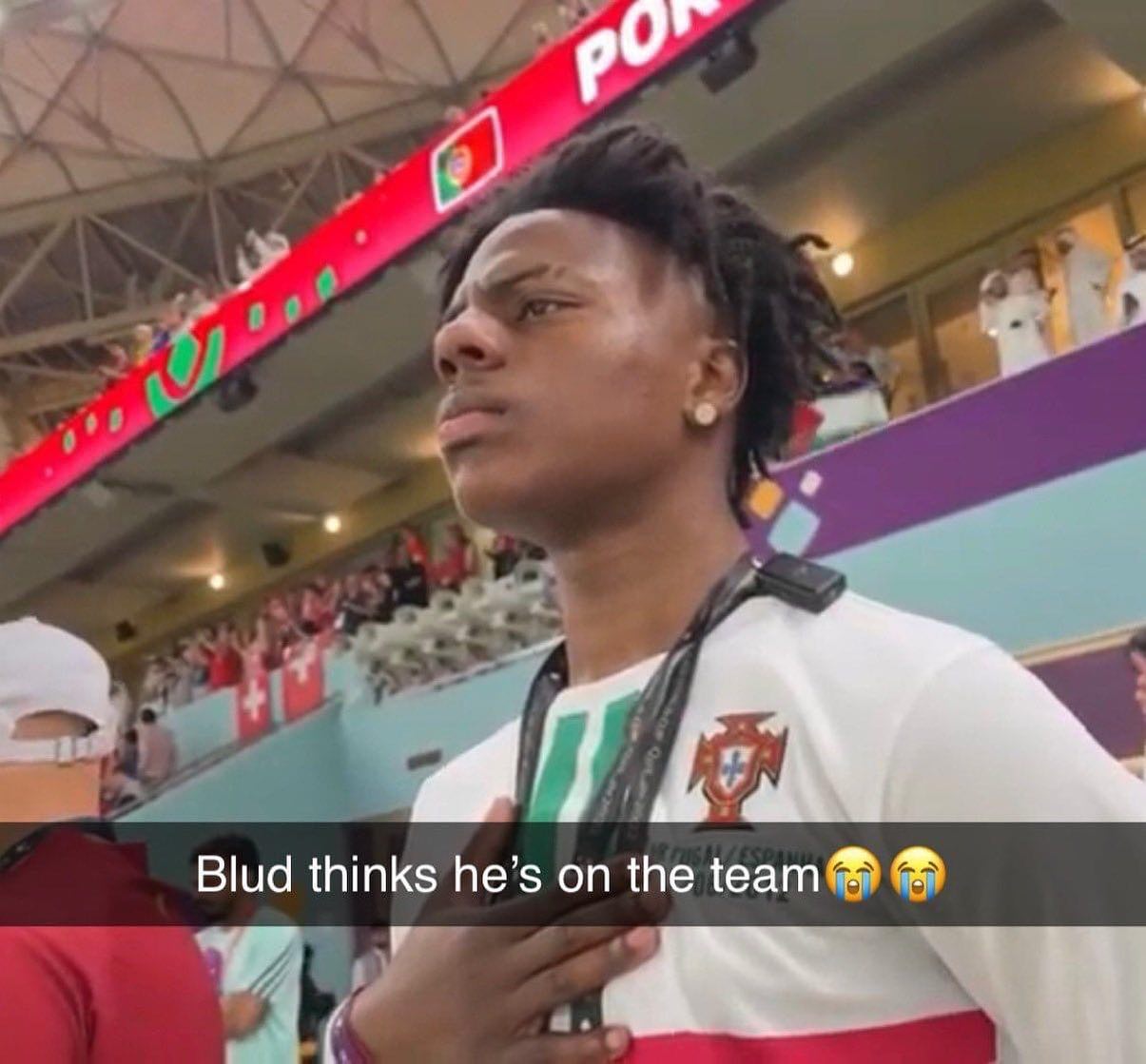
Table of Contents
The rapid advancements in artificial intelligence (AI) have captivated the world, leading many to believe that AI possesses human-like intelligence. However, this perception often overlooks the fundamental differences between AI's "thinking" and true human cognition. While AI has achieved remarkable feats, its cognitive abilities are significantly limited, and understanding these limitations is crucial for responsible development and deployment. This article delves into the key aspects of AI's "thinking," revealing where it falls short of human intelligence.
<h2>AI's Reliance on Data and Algorithms</h2>
AI systems, particularly machine learning models, are fundamentally data-driven. Their ability to perform tasks depends heavily on the quantity and quality of data used for training. This dependence, while enabling impressive performance in specific domains, introduces significant limitations:
-
Bias in data: AI models inherit biases present in their training data, leading to discriminatory or unfair outcomes. For example, an AI system trained on biased facial recognition data may misidentify individuals based on race or gender. This highlights the critical need for diverse and representative datasets.
-
Inability to handle unforeseen situations: AI struggles with "out-of-distribution" data – situations or inputs not encountered during training. A self-driving car trained primarily on sunny-day driving might fail to navigate safely in heavy rain or snow.
-
Lack of generalizability: An AI model trained for image recognition may not be able to perform effectively in natural language processing. This lack of generalizability necessitates training separate models for different tasks, increasing computational costs and data requirements.
These limitations are frequently demonstrated in real-world scenarios. Consider the numerous examples of AI chatbots generating nonsensical or offensive responses due to inadequacies in their training data. The reliance on large datasets also raises concerns about data privacy and security.
<h2>The Absence of True Understanding and Contextual Awareness</h2>
AI, even the most sophisticated systems, lacks genuine understanding. While it excels at pattern recognition, it doesn't possess semantic understanding—the ability to grasp the meaning and context of information. This leads to several crucial limitations:
-
Nuances of language: AI often struggles to interpret nuances in language, such as sarcasm, humor, or metaphors. A simple phrase can be misinterpreted if the AI lacks the contextual understanding required for proper interpretation.
-
Complex social contexts: AI systems find it challenging to navigate complex social interactions, including understanding emotions, intentions, and unspoken cues. This limits their applicability in fields requiring strong social intelligence, such as customer service or healthcare.
-
Pattern recognition vs. comprehension: While AI can identify patterns in data with remarkable accuracy, this does not equate to true comprehension. It might correctly classify an image as a "cat," but it doesn't possess the same understanding of cats as a human does.
Examples of AI misinterpreting human language abound, showcasing the fundamental gap between pattern recognition and true understanding. Improving contextual awareness remains a significant challenge in AI research.
<h2>AI's Lack of Common Sense Reasoning and Emotional Intelligence</h2>
A defining characteristic of human intelligence is common sense reasoning—the ability to apply general knowledge to novel situations. AI largely lacks this ability. It struggles to infer meaning, draw conclusions, or make reasonable assumptions based on limited information. Furthermore, AI generally lacks emotional intelligence:
-
Common sense reasoning: An AI might fail to understand that a wet floor is slippery, even if it has seen thousands of images of wet floors. This highlights the absence of general world knowledge and intuitive reasoning.
-
Emotional intelligence: AI systems typically struggle to recognize, understand, and respond appropriately to human emotions. This is a critical limitation in applications requiring empathy and emotional understanding.
Research in affective computing aims to address this limitation by developing AI systems capable of processing and responding to emotions. However, significant progress is still needed to achieve human-like emotional intelligence.
<h2>The "Black Box" Problem and Explainability</h2>
Many advanced AI systems, particularly deep learning models, operate as "black boxes." Their decision-making processes are opaque and difficult to understand. This lack of transparency poses significant challenges:
-
Understanding decision-making: It's often impossible to determine why an AI system made a specific decision, making it hard to debug errors or build trust.
-
Explainable AI (XAI): The development of XAI techniques is crucial for ensuring transparency and accountability in AI systems. However, creating truly explainable AI models remains a significant research challenge.
The "black box" problem hinders the adoption of AI in high-stakes applications where understanding the reasoning behind decisions is paramount, such as in healthcare and finance.
<h2>Conclusion</h2>
In summary, while AI has made remarkable strides in various domains, its "thinking" abilities are fundamentally limited. Its dependence on vast amounts of data, lack of true understanding and contextual awareness, absence of common sense reasoning and emotional intelligence, and the "black box" problem all represent significant hurdles. AI is a powerful tool, but it is not a replacement for human intelligence. Understanding the limitations of AI "thinking" is crucial for responsible development and deployment. Continue exploring the field to foster informed discussions and ethical practices surrounding artificial cognitive abilities and their impact on society.
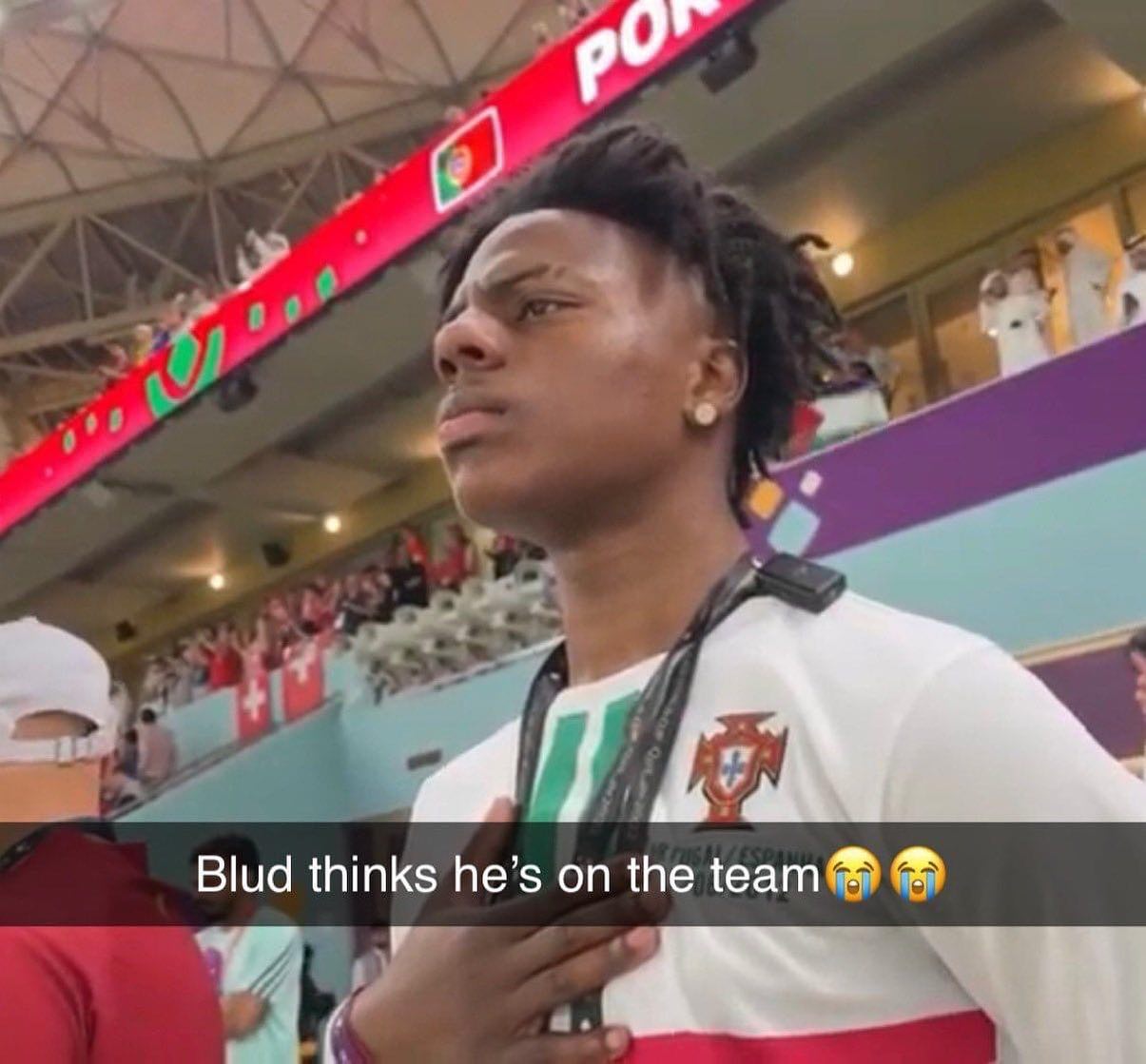
Featured Posts
-
Linda Evans Valentines Day Message A Look At The Dynasty Star At 82
Apr 29, 2025 -
Champions League Der 1 Fc Kaiserslautern Gegen Bayern Muenchen
Apr 29, 2025 -
Netflixs Sirens Trailer Supergirl Milly Alcock And Julianne Moores Cult
Apr 29, 2025 -
Sam Ruddock Missing British Paralympians Disappearance In Las Vegas
Apr 29, 2025 -
April 24 28 Geary County Booking Photos And Records
Apr 29, 2025
Latest Posts
-
Jeff Goldblum And Ariana Grandes Unexpected Collaboration I Dont Know Why I Just Do With The Mildred Snitzer Orchestra
Apr 29, 2025 -
Listen Now Jeff Goldblum Ariana Grande And The Mildred Snitzer Orchestra Release I Dont Know Why I Just Do
Apr 29, 2025 -
Celebrating Culture The Annual Canoe Awakening Event
Apr 29, 2025 -
The Culture Departments Canoe Awakening Traditions And Festivities
Apr 29, 2025 -
Annual Canoe Awakening Celebration A Report From The Culture Department
Apr 29, 2025