The Surprising Simplicity Of AI's "Thinking" Processes
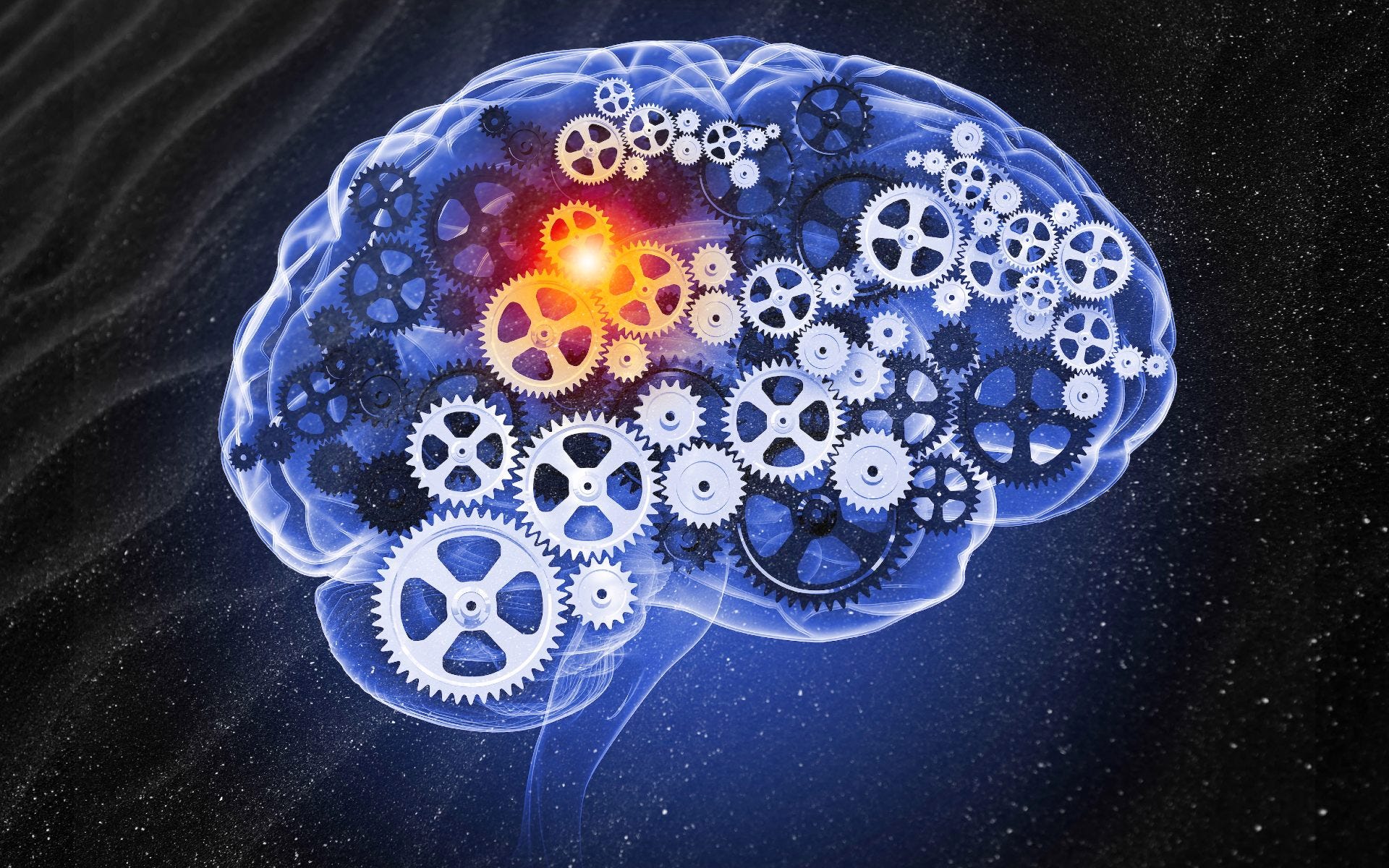
Table of Contents
AI Doesn't "Think" Like Humans: Understanding the Difference
A crucial distinction exists between human cognition and AI's computational processes. While human thought incorporates intuition, emotion, and a lifetime of accumulated experience, AI relies on algorithms, data, and statistical probabilities. AI operates based on pre-programmed rules and learned patterns derived from vast datasets. It doesn't possess consciousness, self-awareness, or genuine understanding in the human sense.
- Humans use intuition, emotion, and experience: We make decisions based on feelings, gut instincts, and past experiences – elements absent in AI.
- AI relies on algorithms, data, and statistical probabilities: AI systems process data through algorithms to identify patterns and predict outcomes based on probability.
- AI operates based on pre-programmed rules and learned patterns: AI's "thinking" is essentially a sophisticated form of pattern recognition and rule application.
This difference highlights the critical distinction between "narrow" or "weak" AI (designed for specific tasks) and the hypothetical "general" or "strong" AI (possessing human-level intelligence), which currently remains largely theoretical. Understanding this distinction is paramount to accurately assessing AI's capabilities and limitations.
The Power of Simple Algorithms: Unveiling the Mechanics
At the heart of AI's functionality lie surprisingly simple algorithms. While the combined effect of millions of these algorithms can seem incredibly complex, the individual components are often elegantly straightforward.
- Machine Learning (ML) Algorithms: Linear regression, for example, finds the best-fitting line through data points to predict future values. Decision trees use a branching structure to classify data based on a series of decisions. These are foundational ML algorithms.
- Neural Networks: These are inspired by the human brain but operate using simple mathematical operations on interconnected nodes. Each node performs a simple calculation, and the network's power comes from the massive number of these simple calculations working in parallel.
- Probability and Statistics: AI heavily relies on statistical methods to analyze data, assess uncertainty, and make predictions. Probability distributions help determine the likelihood of different outcomes.
The simplicity of these algorithms offers significant advantages:
- Simplicity allows for scalability and efficiency: Simple algorithms can be easily implemented on large datasets and processed quickly.
- Simplicity enhances understandability and debugging: Simpler systems are easier to analyze and troubleshoot.
- Simplicity doesn't equate to lack of sophistication: The combination of numerous simple algorithms can create highly sophisticated AI systems capable of complex tasks.
Data is King: How AI Learns and Improves
Data is the lifeblood of AI. AI models are trained on massive datasets, learning to recognize patterns and make predictions based on this information. The training process involves feeding the AI model data and adjusting its parameters to minimize errors. This iterative refinement process leads to improved accuracy and performance.
- The more data, the better the AI model performs: Larger datasets typically lead to more robust and accurate AI models.
- Data quality is paramount for accurate and reliable AI: Inaccurate or biased data will result in an AI model that reflects those flaws.
- Data preprocessing and cleaning are essential steps: Raw data often needs cleaning and transformation before it can be used to train an AI model.
Different machine learning paradigms utilize data in various ways:
- Supervised learning: The algorithm learns from labeled data, where each data point is associated with a known outcome.
- Unsupervised learning: The algorithm identifies patterns and structures in unlabeled data without predefined outcomes.
- Reinforcement learning: The algorithm learns through trial and error, receiving rewards or penalties for its actions.
Limitations of Simple AI: Addressing the Challenges
Despite their power, current AI systems have significant limitations stemming from their reliance on data and algorithms.
- Bias in data can lead to biased AI outputs: If the training data reflects societal biases, the AI model will likely perpetuate these biases.
- AI struggles with tasks requiring common sense and reasoning: AI excels at pattern recognition but often lacks the contextual understanding and common sense that humans possess.
- AI's lack of creativity and true understanding poses limitations: AI can generate creative outputs, but it doesn't genuinely understand the concepts it manipulates.
Ethical considerations are paramount in AI development. Mitigating bias, ensuring transparency, and promoting responsible AI development are crucial to harnessing AI's potential while minimizing its risks.
Conclusion: The Surprising Simplicity and Future of AI's "Thinking" Processes
AI's "thinking" is fundamentally based on surprisingly simple algorithms, data, and statistical models. This simplicity underlies its power and scalability, yet also explains its inherent limitations. The core difference between human and AI "thinking" lies in the absence of consciousness, intuition, and genuine understanding in artificial systems. Understanding the surprising simplicity of AI's 'thinking' processes is the first step to navigating the exciting future of this rapidly evolving technology. Explore further and uncover the fascinating mechanics behind AI's decision-making today!
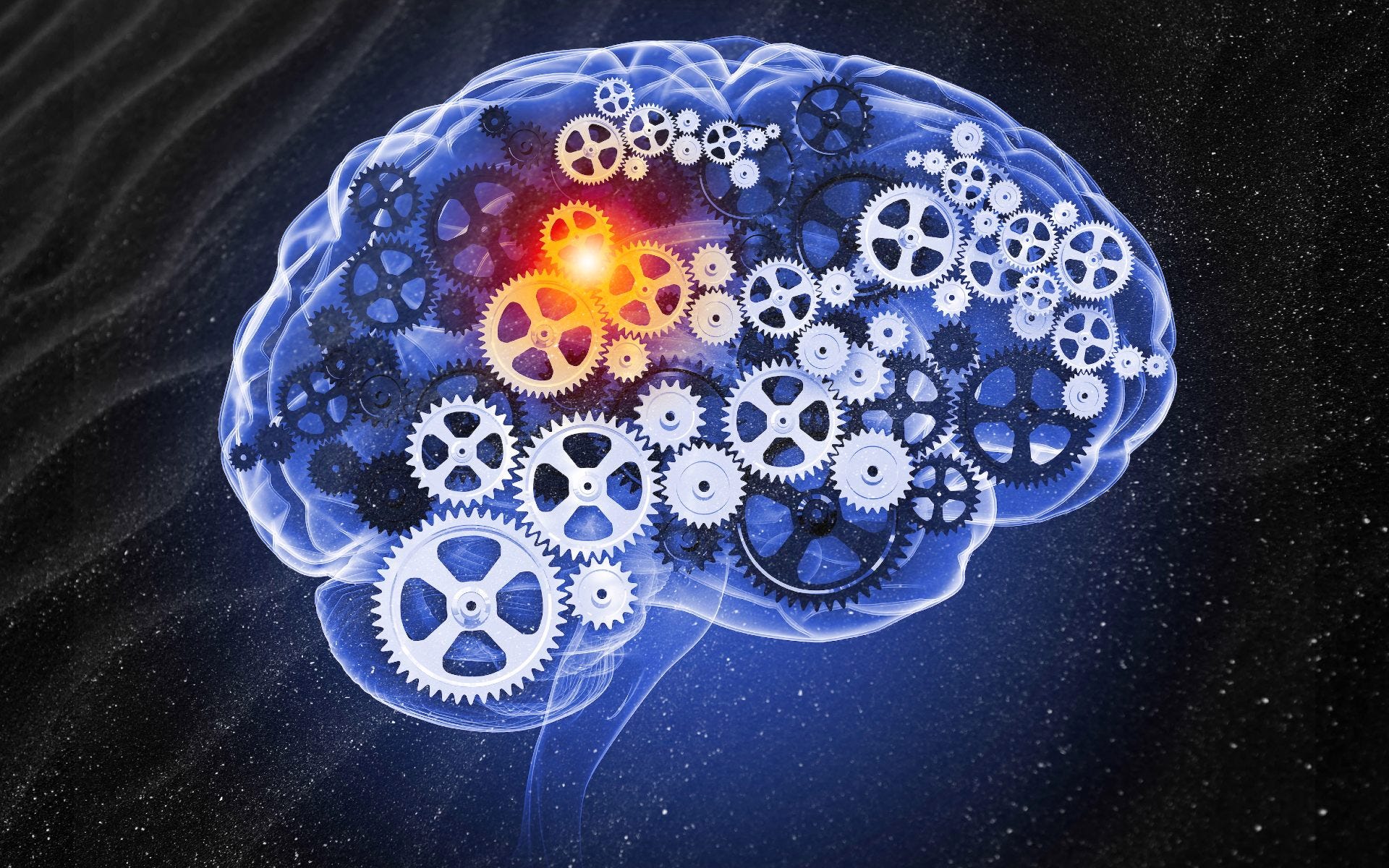
Featured Posts
-
The Unexpected Rise Of Macario Martinez From Street Sweeper To National Fame
Apr 29, 2025 -
Exclusive University Group Challenges Trump Administration Policies
Apr 29, 2025 -
Open Thread Community February 16 2025 Updates
Apr 29, 2025 -
Rosenberg Critiques Bank Of Canadas Cautious Approach
Apr 29, 2025 -
Recent Russian Military Activities A Cause For European Concern
Apr 29, 2025
Latest Posts
-
Convicted Cardinals Vote A Key Issue In The Upcoming Papal Conclave
Apr 29, 2025 -
Convicted Cardinals Right To Vote In Papal Conclave
Apr 29, 2025 -
Papal Conclave Debate Over Convicted Cardinals Voting Eligibility
Apr 29, 2025 -
Convicted Cardinal Claims Entitlement To Vote In Next Papal Election
Apr 29, 2025 -
Cardinals Conviction And Papal Conclave Voting Eligibility
Apr 29, 2025